Linear programming (LP), also called linear optimization, is a method to achieve the best outcome (such as maximum profit or lowest cost) in a mathematical model whose requirements are represented by linear relationships. Linear programming is a special case of mathematical programming (also known as mathematical optimization).
More formally, linear programming is a technique for the optimization of a linear objective function, subject to linear equality and linear inequality constraints. Its feasible region is a convex polytope, which is a set defined as the intersection of finitely many half spaces, each of which is defined by a linear inequality. Its objective function is a real-valued affine (linear) function defined on this polyhedron. A linear programming algorithm finds a point in the polytope where this function has the smallest (or largest) value if such a point exists.
Linear programs are problems that can be expressed in canonical form as
Here the components of x are the variables to be determined, c and b are given vectors (with indicating that the coefficients of c are used as a single-row matrix for the purpose of forming the matrix product), and A is a given matrix. The function whose value is to be maximized or minimized ( in this case) is called the objective function. The inequalities Ax ≤ b and x ≥ 0 are the constraints which specify a convex polytope over which the objective function is to be optimized. In this context, two vectors are comparable when they have the same dimensions. If every entry in the first is less-than or equal-to the corresponding entry in the second, then it can be said that the first vector is less-than or equal-to the second vector.
Linear programming can be applied to various fields of study. It is widely used in mathematics and, to a lesser extent, in business, economics, and some engineering problems. Industries that use linear programming models include transportation, energy, telecommunications, and manufacturing. It has proven useful in modeling diverse types of problems in planning, routing, scheduling, assignment, and design.
History
The problem of solving a system of linear inequalities dates back at least as far as Fourier, who in 1827 published a method for solving them, and after whom the method of Fourier–Motzkin elimination is named.
In 1939 a linear programming formulation of a problem that is equivalent to the general linear programming problem was given by the Soviet mathematician and economist Leonid Kantorovich, who also proposed a method for solving it. It is a way he developed, during World War II, to plan expenditures and returns in order to reduce costs of the army and to increase losses imposed on the enemy. Kantorovich's work was initially neglected in the USSR. About the same time as Kantorovich, the Dutch-American economist T. C. Koopmans formulated classical economic problems as linear programs. Kantorovich and Koopmans later shared the 1975 Nobel prize in economics. In 1941, Frank Lauren Hitchcock also formulated transportation problems as linear programs and gave a solution very similar to the later simplex method. Hitchcock had died in 1957, and the Nobel prize is not awarded posthumously.
From 1946 to 1947 George B. Dantzig independently developed general linear programming formulation to use for planning problems in the US Air Force. In 1947, Dantzig also invented the simplex method that, for the first time efficiently, tackled the linear programming problem in most cases. When Dantzig arranged a meeting with John von Neumann to discuss his simplex method, Neumann immediately conjectured the theory of duality by realizing that the problem he had been working in game theory was equivalent. Dantzig provided formal proof in an unpublished report "A Theorem on Linear Inequalities" on January 5, 1948. Dantzig's work was made available to public in 1951. In the post-war years, many industries applied it in their daily planning.
Dantzig's original example was to find the best assignment of 70 people to 70 jobs. The computing power required to test all the permutations to select the best assignment is vast; the number of possible configurations exceeds the number of particles in the observable universe. However, it takes only a moment to find the optimum solution by posing the problem as a linear program and applying the simplex algorithm. The theory behind linear programming drastically reduces the number of possible solutions that must be checked.
The linear programming problem was first shown to be solvable in polynomial time by Leonid Khachiyan in 1979, but a larger theoretical and practical breakthrough in the field came in 1984 when Narendra Karmarkar introduced a new interior-point method for solving linear-programming problems.
Uses
Linear programming is a widely used field of optimization for several reasons. Many practical problems in operations research can be expressed as linear programming problems. Certain special cases of linear programming, such as network flow problems and multicommodity flow problems, are considered important enough to have much research on specialized algorithms. A number of algorithms for other types of optimization problems work by solving linear programming problems as sub-problems. Historically, ideas from linear programming have inspired many of the central concepts of optimization theory, such as duality, decomposition, and the importance of convexity and its generalizations. Likewise, linear programming was heavily used in the early formation of microeconomics, and it is currently utilized in company management, such as planning, production, transportation, and technology. Although the modern management issues are ever-changing, most companies would like to maximize profits and minimize costs with limited resources. Google also uses linear programming to stabilize YouTube videos.
Standard form
Standard form is the usual and most intuitive form of describing a linear programming problem. It consists of the following three parts:
- A linear function to be maximized
- e.g.
- Problem constraints of the following form
- e.g.
- Non-negative variables
- e.g.
The problem is usually expressed in matrix form, and then becomes:
Other forms, such as minimization problems, problems with constraints on alternative forms, and problems involving negative variables can always be rewritten into an equivalent problem in standard form.
Example
Suppose that a farmer has a piece of farm land, say L km2, to be planted with either wheat or barley or some combination of the two. The farmer has a limited amount of fertilizer, F kilograms, and pesticide, P kilograms. Every square kilometer of wheat requires F1 kilograms of fertilizer and P1 kilograms of pesticide, while every square kilometer of barley requires F2 kilograms of fertilizer and P2 kilograms of pesticide. Let S1 be the selling price of wheat per square kilometer, and S2 be the selling price of barley. If we denote the area of land planted with wheat and barley by x1 and x2 respectively, then profit can be maximized by choosing optimal values for x1 and x2. This problem can be expressed with the following linear programming problem in the standard form:
Maximize: | (maximize the revenue (the total wheat sales plus the total barley sales) – revenue is the "objective function") | |
Subject to: | (limit on total area) | |
|
(limit on fertilizer) | |
|
(limit on pesticide) | |
|
(cannot plant a negative area). |
In matrix form this becomes:
- maximize
- subject to
Augmented form (slack form)
Linear programming problems can be converted into an augmented form in order to apply the common form of the simplex algorithm. This form introduces non-negative slack variables to replace inequalities with equalities in the constraints. The problems can then be written in the following block matrix form:
- Maximize :
where are the newly introduced slack variables, are the decision variables, and is the variable to be maximized.
Example
The example above is converted into the following augmented form:
Maximize: (objective function) subject to: (augmented constraint) (augmented constraint) (augmented constraint)
where are (non-negative) slack variables, representing in this example the unused area, the amount of unused fertilizer, and the amount of unused pesticide.
In matrix form this becomes:
- Maximize :
Duality
Every linear programming problem, referred to as a primal problem, can be converted into a dual problem, which provides an upper bound to the optimal value of the primal problem. In matrix form, we can express the primal problem as:
- Maximize cTx subject to Ax ≤ b, x ≥ 0;
- with the corresponding symmetric dual problem,
- Minimize bTy subject to ATy ≥ c, y ≥ 0.
An alternative primal formulation is:
- Maximize cTx subject to Ax ≤ b;
- with the corresponding asymmetric dual problem,
- Minimize bTy subject to ATy = c, y ≥ 0.
There are two ideas fundamental to duality theory. One is the fact that (for the symmetric dual) the dual of a dual linear program is the original primal linear program. Additionally, every feasible solution for a linear program gives a bound on the optimal value of the objective function of its dual. The weak duality theorem states that the objective function value of the dual at any feasible solution is always greater than or equal to the objective function value of the primal at any feasible solution. The strong duality theorem states that if the primal has an optimal solution, x*, then the dual also has an optimal solution, y*, and cTx*=bTy*.
A linear program can also be unbounded or infeasible. Duality theory tells us that if the primal is unbounded then the dual is infeasible by the weak duality theorem. Likewise, if the dual is unbounded, then the primal must be infeasible. However, it is possible for both the dual and the primal to be infeasible. See dual linear program for details and several more examples.
Variations
Covering/packing dualities
A covering LP is a linear program of the form:
- Minimize: bTy,
- subject to: ATy ≥ c, y ≥ 0,
such that the matrix A and the vectors b and c are non-negative.
The dual of a covering LP is a packing LP, a linear program of the form:
- Maximize: cTx,
- subject to: Ax ≤ b, x ≥ 0,
such that the matrix A and the vectors b and c are non-negative.
Examples
Covering and packing LPs commonly arise as a linear programming relaxation of a combinatorial problem and are important in the study of approximation algorithms. For example, the LP relaxations of the set packing problem, the independent set problem, and the matching problem are packing LPs. The LP relaxations of the set cover problem, the vertex cover problem, and the dominating set problem are also covering LPs.
Finding a fractional coloring of a graph is another example of a covering LP. In this case, there is one constraint for each vertex of the graph and one variable for each independent set of the graph.
Complementary slackness
It is possible to obtain an optimal solution to the dual when only an optimal solution to the primal is known using the complementary slackness theorem. The theorem states:
Suppose that x = (x1, x2, ... , xn) is primal feasible and that y = (y1, y2, ... , ym) is dual feasible. Let (w1, w2, ..., wm) denote the corresponding primal slack variables, and let (z1, z2, ... , zn) denote the corresponding dual slack variables. Then x and y are optimal for their respective problems if and only if
- xj zj = 0, for j = 1, 2, ... , n, and
- wi yi = 0, for i = 1, 2, ... , m.
So if the i-th slack variable of the primal is not zero, then the i-th variable of the dual is equal to zero. Likewise, if the j-th slack variable of the dual is not zero, then the j-th variable of the primal is equal to zero.
This necessary condition for optimality conveys a fairly simple economic principle. In standard form (when maximizing), if there is slack in a constrained primal resource (i.e., there are "leftovers"), then additional quantities of that resource must have no value. Likewise, if there is slack in the dual (shadow) price non-negativity constraint requirement, i.e., the price is not zero, then there must be scarce supplies (no "leftovers").
Theory
Existence of optimal solutions
Geometrically, the linear constraints define the feasible region, which is a convex polyhedron. A linear function is a convex function, which implies that every local minimum is a global minimum; similarly, a linear function is a concave function, which implies that every local maximum is a global maximum.
An optimal solution need not exist, for two reasons. First, if the constraints are inconsistent, then no feasible solution exists: For instance, the constraints x ≥ 2 and x ≤ 1 cannot be satisfied jointly; in this case, we say that the LP is infeasible. Second, when the polytope is unbounded in the direction of the gradient of the objective function (where the gradient of the objective function is the vector of the coefficients of the objective function), then no optimal value is attained because it is always possible to do better than any finite value of the objective function.
Optimal vertices (and rays) of polyhedra
Otherwise, if a feasible solution exists and if the constraint set is bounded, then the optimum value is always attained on the boundary of the constraint set, by the maximum principle for convex functions (alternatively, by the minimum principle for concave functions) since linear functions are both convex and concave. However, some problems have distinct optimal solutions; for example, the problem of finding a feasible solution to a system of linear inequalities is a linear programming problem in which the objective function is the zero function (that is, the constant function taking the value zero everywhere). For this feasibility problem with the zero-function for its objective-function, if there are two distinct solutions, then every convex combination of the solutions is a solution.
The vertices of the polytope are also called basic feasible solutions. The reason for this choice of name is as follows. Let d denote the number of variables. Then the fundamental theorem of linear inequalities implies (for feasible problems) that for every vertex x* of the LP feasible region, there exists a set of d (or fewer) inequality constraints from the LP such that, when we treat those d constraints as equalities, the unique solution is x*. Thereby we can study these vertices by means of looking at certain subsets of the set of all constraints (a discrete set), rather than the continuum of LP solutions. This principle underlies the simplex algorithm for solving linear programs.
Algorithms
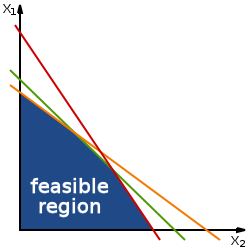
Basis exchange algorithms
Simplex algorithm of Dantzig
The simplex algorithm, developed by George Dantzig in 1947, solves LP problems by constructing a feasible solution at a vertex of the polytope and then walking along a path on the edges of the polytope to vertices with non-decreasing values of the objective function until an optimum is reached for sure. In many practical problems, "stalling" occurs: many pivots are made with no increase in the objective function. In rare practical problems, the usual versions of the simplex algorithm may actually "cycle". To avoid cycles, researchers developed new pivoting rules.
In practice, the simplex algorithm is quite efficient and can be guaranteed to find the global optimum if certain precautions against cycling are taken. The simplex algorithm has been proved to solve "random" problems efficiently, i.e. in a cubic number of steps, which is similar to its behavior on practical problems.
However, the simplex algorithm has poor worst-case behavior: Klee and Minty constructed a family of linear programming problems for which the simplex method takes a number of steps exponential in the problem size. In fact, for some time it was not known whether the linear programming problem was solvable in polynomial time, i.e. of complexity class P.
Criss-cross algorithm
Like the simplex algorithm of Dantzig, the criss-cross algorithm is a basis-exchange algorithm that pivots between bases. However, the criss-cross algorithm need not maintain feasibility, but can pivot rather from a feasible basis to an infeasible basis. The criss-cross algorithm does not have polynomial time-complexity for linear programming. Both algorithms visit all 2D corners of a (perturbed) cube in dimension D, the Klee–Minty cube, in the worst case.
Interior point
In contrast to the simplex algorithm, which finds an optimal solution by traversing the edges between vertices on a polyhedral set, interior-point methods move through the interior of the feasible region.
Ellipsoid algorithm, following Khachiyan
This is the first worst-case polynomial-time algorithm ever found for linear programming. To solve a problem which has n variables and can be encoded in L input bits, this algorithm runs in time. Leonid Khachiyan solved this long-standing complexity issue in 1979 with the introduction of the ellipsoid method. The convergence analysis has (real-number) predecessors, notably the iterative methods developed by Naum Z. Shor and the approximation algorithms by Arkadi Nemirovski and D. Yudin.
Projective algorithm of Karmarkar
Khachiyan's algorithm was of landmark importance for establishing the polynomial-time solvability of linear programs. The algorithm was not a computational break-through, as the simplex method is more efficient for all but specially constructed families of linear programs.
However, Khachiyan's algorithm inspired new lines of research in linear programming. In 1984, N. Karmarkar proposed a projective method for linear programming. Karmarkar's algorithm improved on Khachiyan's worst-case polynomial bound (giving ). Karmarkar claimed that his algorithm was much faster in practical LP than the simplex method, a claim that created great interest in interior-point methods. Since Karmarkar's discovery, many interior-point methods have been proposed and analyzed.
Vaidya's 87 algorithm
In 1987, Vaidya proposed an algorithm that runs in time.
Vaidya's 89 algorithm
In 1989, Vaidya developed an algorithm that runs in time. Formally speaking, the algorithm takes arithmetic operations in the worst case, where is the number of constraints, is the number of variables, and is the number of bits.
Input sparsity time algorithms
In 2015, Lee and Sidford showed that, it can be solved in time, where represents the number of non-zero elements, and it remains taking in the worst case.
Current matrix multiplication time algorithm
In 2019, Cohen, Lee and Song improved the running time to time, is the exponent of matrix multiplication and is the dual exponent of matrix multiplication. is (roughly) defined to be the largest number such that one can multiply an matrix by a matrix in time. In a followup work by Lee, Song and Zhang, they reproduce the same result via a different method. These two algorithms remain when and . The result due to Jiang, Song, Weinstein and Zhang improved to .
Comparison of interior-point methods and simplex algorithms
The current opinion is that the efficiencies of good implementations of simplex-based methods and interior point methods are similar for routine applications of linear programming. However, for specific types of LP problems, it may be that one type of solver is better than another (sometimes much better), and that the structure of the solutions generated by interior point methods versus simplex-based methods are significantly different with the support set of active variables being typically smaller for the latter one.
Open problems and recent work
Does linear programming admit a strongly polynomial-time algorithm?
There are several open problems in the theory of linear programming, the solution of which would represent fundamental breakthroughs in mathematics and potentially major advances in our ability to solve large-scale linear programs.
- Does LP admit a strongly polynomial-time algorithm?
- Does LP admit a strongly polynomial-time algorithm to find a strictly complementary solution?
- Does LP admit a polynomial-time algorithm in the real number (unit cost) model of computation?
This closely related set of problems has been cited by Stephen Smale as among the 18 greatest unsolved problems of the 21st century. In Smale's words, the third version of the problem "is the main unsolved problem of linear programming theory." While algorithms exist to solve linear programming in weakly polynomial time, such as the ellipsoid methods and interior-point techniques, no algorithms have yet been found that allow strongly polynomial-time performance in the number of constraints and the number of variables. The development of such algorithms would be of great theoretical interest, and perhaps allow practical gains in solving large LPs as well.
Although the Hirsch conjecture was recently disproved for higher dimensions, it still leaves the following questions open.
- Are there pivot rules which lead to polynomial-time simplex variants?
- Do all polytopal graphs have polynomially bounded diameter?
These questions relate to the performance analysis and development of simplex-like methods. The immense efficiency of the simplex algorithm in practice despite its exponential-time theoretical performance hints that there may be variations of simplex that run in polynomial or even strongly polynomial time. It would be of great practical and theoretical significance to know whether any such variants exist, particularly as an approach to deciding if LP can be solved in strongly polynomial time.
The simplex algorithm and its variants fall in the family of edge-following algorithms, so named because they solve linear programming problems by moving from vertex to vertex along edges of a polytope. This means that their theoretical performance is limited by the maximum number of edges between any two vertices on the LP polytope. As a result, we are interested in knowing the maximum graph-theoretical diameter of polytopal graphs. It has been proved that all polytopes have subexponential diameter. The recent disproof of the Hirsch conjecture is the first step to prove whether any polytope has superpolynomial diameter. If any such polytopes exist, then no edge-following variant can run in polynomial time. Questions about polytope diameter are of independent mathematical interest.
Simplex pivot methods preserve primal (or dual) feasibility. On the other hand, criss-cross pivot methods do not preserve (primal or dual) feasibility – they may visit primal feasible, dual feasible or primal-and-dual infeasible bases in any order. Pivot methods of this type have been studied since the 1970s. Essentially, these methods attempt to find the shortest pivot path on the arrangement polytope under the linear programming problem. In contrast to polytopal graphs, graphs of arrangement polytopes are known to have small diameter, allowing the possibility of strongly polynomial-time criss-cross pivot algorithm without resolving questions about the diameter of general polytopes.
Integer unknowns
If all of the unknown variables are required to be integers, then the problem is called an integer programming (IP) or integer linear programming (ILP) problem. In contrast to linear programming, which can be solved efficiently in the worst case, integer programming problems are in many practical situations (those with bounded variables) NP-hard. 0–1 integer programming or binary integer programming (BIP) is the special case of integer programming where variables are required to be 0 or 1 (rather than arbitrary integers). This problem is also classified as NP-hard, and in fact the decision version was one of Karp's 21 NP-complete problems.
If only some of the unknown variables are required to be integers, then the problem is called a mixed integer (linear) programming (MIP or MILP) problem. These are generally also NP-hard because they are even more general than ILP programs.
There are however some important subclasses of IP and MIP problems that are efficiently solvable, most notably problems where the constraint matrix is totally unimodular and the right-hand sides of the constraints are integers or – more general – where the system has the total dual integrality (TDI) property.
Advanced algorithms for solving integer linear programs include:
- cutting-plane method
- Branch and bound
- Branch and cut
- Branch and price
- if the problem has some extra structure, it may be possible to apply delayed column generation.
Such integer-programming algorithms are discussed by Padberg and in Beasley.
Integral linear programs
A linear program in real variables is said to be integral if it has at least one optimal solution which is integral, i.e., made of only integer values. Likewise, a polyhedron is said to be integral if for all bounded feasible objective functions c, the linear program has an optimum with integer coordinates. As observed by Edmonds and Giles in 1977, one can equivalently say that the polyhedron is integral if for every bounded feasible integral objective function c, the optimal value of the linear program is an integer.
Integral linear programs are of central importance in the polyhedral aspect of combinatorial optimization since they provide an alternate characterization of a problem. Specifically, for any problem, the convex hull of the solutions is an integral polyhedron; if this polyhedron has a nice/compact description, then we can efficiently find the optimal feasible solution under any linear objective. Conversely, if we can prove that a linear programming relaxation is integral, then it is the desired description of the convex hull of feasible (integral) solutions.
Terminology is not consistent throughout the literature, so one should be careful to distinguish the following two concepts,
- in an integer linear program, described in the previous section, variables are forcibly constrained to be integers, and this problem is NP-hard in general,
- in an integral linear program, described in this section, variables are not constrained to be integers but rather one has proven somehow that the continuous problem always has an integral optimal value (assuming c is integral), and this optimal value may be found efficiently since all polynomial-size linear programs can be solved in polynomial time.
One common way of proving that a polyhedron is integral is to show that it is totally unimodular. There are other general methods including the integer decomposition property and total dual integrality. Other specific well-known integral LPs include the matching polytope, lattice polyhedra, submodular flow polyhedra, and the intersection of two generalized polymatroids/g-polymatroids – e.g. see Schrijver 2003.
Solvers and scripting (programming) languages
Permissive licenses:
Name | License | Brief info |
---|---|---|
Gekko | MIT License | Open-source library for solving large-scale LP, QP, QCQP, NLP, and MIP optimization |
GLOP | Apache v2 | Google's open-source linear programming solver |
Pyomo | BSD | An open-source modeling language for large-scale linear, mixed integer and nonlinear optimization |
SuanShu | Apache v2 | an open-source suite of optimization algorithms to solve LP, QP, SOCP, SDP, SQP in Java |
Copyleft (reciprocal) licenses:
Name | License | Brief info |
---|---|---|
ALGLIB | GPL 2+ | an LP solver from ALGLIB project (C++, C#, Python) |
Cassowary constraint solver | LGPL | an incremental constraint solving toolkit that efficiently solves systems of linear equalities and inequalities |
CLP | CPL | an LP solver from COIN-OR |
glpk | GPL | GNU Linear Programming Kit, an LP/MILP solver with a native C API and numerous (15) third-party wrappers for other languages. Specialist support for flow networks. Bundles the AMPL-like GNU MathProg modelling language and translator. |
Qoca | GPL | a library for incrementally solving systems of linear equations with various goal functions |
R-Project | GPL | a programming language and software environment for statistical computing and graphics |
MINTO (Mixed Integer Optimizer, an integer programming solver which uses branch and bound algorithm) has publicly available source code but is not open source.
Proprietary licenses:
Name | Brief info |
---|---|
AIMMS | A modeling language that allows to model linear, mixed integer, and nonlinear optimization models. It also offers a tool for constraint programming. Algorithm, in the forms of heuristics or exact methods, such as Branch-and-Cut or Column Generation, can also be implemented. The tool calls an appropriate solver such as CPLEX or similar, to solve the optimization problem at hand. Academic licenses are free of charge. |
ALGLIB | A commercial edition of the copyleft licensed library. C++, C#, Python. |
AMPL | A popular modeling language for large-scale linear, mixed integer and nonlinear optimisation with a free student limited version available (500 variables and 500 constraints). |
Analytica | A general modeling language and interactive development environment. Its influence diagrams enable users to formulate problems as graphs with nodes for decision variables, objectives, and constraints. Analytica Optimizer Edition includes linear, mixed integer, and nonlinear solvers and selects the solver to match the problem. It also accepts other engines as plug-ins, including XPRESS, Gurobi, Artelys Knitro, and MOSEK. |
APMonitor | API to MATLAB and Python. Solve example Linear Programming (LP) problems through MATLAB, Python, or a web-interface. |
CPLEX | Popular solver with an API for several programming languages, and also has a modelling language and works with AIMMS, AMPL, GAMS, MPL, OpenOpt, OPL Development Studio, and TOMLAB. Free for academic use. |
Excel Solver Function | A nonlinear solver adjusted to spreadsheets in which function evaluations are based on the recalculating cells. Basic version available as a standard add-on for Excel. |
FortMP |
|
GAMS |
|
IMSL Numerical Libraries | Collections of math and statistical algorithms available in C/C++, Fortran, Java and C#/.NET. Optimization routines in the IMSL Libraries include unconstrained, linearly and nonlinearly constrained minimizations, and linear programming algorithms. |
LINDO | Solver with an API for large scale optimization of linear, integer, quadratic, conic and general nonlinear programs with stochastic programming extensions. It offers a global optimization procedure for finding guaranteed globally optimal solution to general nonlinear programs with continuous and discrete variables. It also has a statistical sampling API to integrate Monte-Carlo simulations into an optimization framework. It has an algebraic modeling language (LINGO) and allows modeling within a spreadsheet (What'sBest). |
Maple | A general-purpose programming-language for symbolic and numerical computing. |
MATLAB | A general-purpose and matrix-oriented programming-language for numerical computing. Linear programming in MATLAB requires the Optimization Toolbox in addition to the base MATLAB product; available routines include INTLINPROG and LINPROG |
Mathcad | A WYSIWYG math editor. It has functions for solving both linear and nonlinear optimization problems. |
Mathematica | A general-purpose programming-language for mathematics, including symbolic and numerical capabilities. |
MOSEK | A solver for large scale optimization with API for several languages (C++,java,.net, Matlab and python). |
NAG Numerical Library | A collection of mathematical and statistical routines developed by the Numerical Algorithms Group for multiple programming languages (C, C++, Fortran, Visual Basic, Java and C#) and packages (MATLAB, Excel, R, LabVIEW). The Optimization chapter of the NAG Library includes routines for linear programming problems with both sparse and non-sparse linear constraint matrices, together with routines for the optimization of quadratic, nonlinear, sums of squares of linear or nonlinear functions with nonlinear, bounded or no constraints. The NAG Library has routines for both local and global optimization, and for continuous or integer problems. |
OptimJ | A Java-based modeling language for optimization with a free version available.[27][28] |
SAS/OR | A suite of solvers for Linear, Integer, Nonlinear, Derivative-Free, Network, Combinatorial and Constraint Optimization; the Algebraic modeling language OPTMODEL; and a variety of vertical solutions aimed at specific problems/markets, all of which are fully integrated with the SAS System. |
SCIP | A general-purpose constraint integer programming solver with an emphasis on MIP. Compatible with Zimpl modelling language. Free for academic use and available in source code. |
XPRESS | Solver for large-scale linear programs, quadratic programs, general nonlinear and mixed-integer programs. Has API for several programming languages, also has a modelling language Mosel and works with AMPL, GAMS. Free for academic use. |
VisSim | A visual block diagram language for simulation of dynamical systems. |