What would it take for artificial intelligence to make real progress?
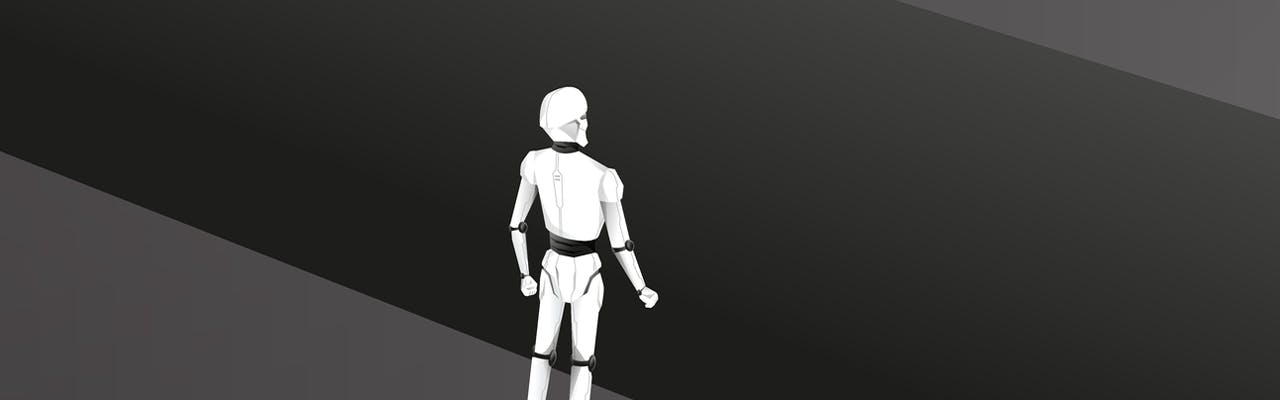
Let me start by saying a few things that seem obvious,” Geoffrey Hinton, “Godfather” of deep learning, and one of the most celebrated scientists of our time, told a leading AI conference in Toronto in 2016. “If you work as a radiologist you’re like the coyote that’s already over the edge of the cliff but hasn’t looked down.” Deep learning is so well-suited to reading images from MRIs and CT scans, he reasoned, that people should “stop training radiologists now” and that it’s “just completely obvious within five years deep learning is going to do better.”
Fast forward to 2022, and not a single radiologist has been replaced. Rather, the consensus view nowadays is that machine learning for radiology is harder than it looks1; at least for now, humans and machines complement each other’s strengths.2
Deep learning is at its best when all we need are rough-ready results.
Few fields have been more filled with hype and bravado than artificial intelligence. It has flitted from fad to fad decade by decade, always promising the moon, and only occasionally delivering. One minute it was expert systems, next it was Bayesian networks, and then Support Vector Machines. In 2011, it was IBM’s Watson, once pitched as a revolution in medicine, more recently sold for parts.3 Nowadays, and in fact ever since 2012, the flavor of choice has been deep learning, the multibillion-dollar technique that drives so much of contemporary AI and which Hinton helped pioneer: He’s been cited an astonishing half-million times and won, with Yoshua Bengio and Yann LeCun, the 2018 Turing Award.
Like AI pioneers before him, Hinton frequently heralds the Great Revolution that is coming. Radiology is just part of it. In 2015, shortly after Hinton joined Google, The Guardian reported that the company was on the verge of “developing algorithms with the capacity for logic, natural conversation and even flirtation.” In November 2020, Hinton told MIT Technology Review that “deep learning is going to be able to do everything.”4
I seriously doubt it. In truth, we are still a long way from machines that can genuinely understand human language, and nowhere near the ordinary day-to-day intelligence of Rosey the Robot, a science-fiction housekeeper that could not only interpret a wide variety of human requests but safely act on them in real time. Sure, Elon Musk recently said that the new humanoid robot he was hoping to build, Optimus, would someday be bigger than the vehicle industry, but as of Tesla’s AI Demo Day 2021, in which the robot was announced, Optimus was nothing more than a human in a costume. Google’s latest contribution to language is a system (Lamda) that is so flighty that one of its own authors recently acknowledged it is prone to producing “bullshit.”5 Turning the tide, and getting to AI we can really trust, ain’t going to be easy.
In time we will see that deep learning was only a tiny part of what we need to build if we’re ever going to get trustworthy AI.
Deep learning, which is fundamentally a technique for recognizing patterns, is at its best when all we need are rough-ready results, where stakes are low and perfect results optional. Take photo tagging. I asked my iPhone the other day to find a picture of a rabbit that I had taken a few years ago; the phone obliged instantly, even though I never labeled the picture. It worked because my rabbit photo was similar enough to other photos in some large database of other rabbit-labeled photos. But automatic, deep-learning-powered photo tagging is also prone to error; it may miss some rabbit photos (especially cluttered ones, or ones taken with weird light or unusual angles or with the rabbit partly obscured; it occasionally confuses baby photos of my two children. But the stakes are low—if the app makes an occasional error, I am not going to throw away my phone.
When the stakes are higher, though, as in radiology or driverless cars, we need to be much more cautious about adopting deep learning. When a single error can cost a life, it’s just not good enough. Deep-learning systems are particularly problematic when it comes to “outliers” that differ substantially from the things on which they are trained. Not long ago, for example, a Tesla in so-called “Full Self Driving Mode” encountered a person holding up a stop sign in the middle of a road. The car failed to recognize the person (partly obscured by the stop sign) and the stop sign (out of its usual context on the side of a road); the human driver had to take over. The scene was far enough outside of the training database that the system had no idea what to do.
Few fields have been more filled with hype than artificial intelligence.
Current deep-learning systems frequently succumb to stupid errors like this. They sometimes misread dirt on an image that a human radiologist would recognize as a glitch. (Another issue for radiology systems, and key motivation for keeping humans in the loop, is that current AI relies mostly or entirely on images, with little or no comprehension of all the text that might describe a patient’s history, sometimes neglecting critical information.) A deep-learning system has mislabeled an apple as an iPod because the apple had a piece of paper in front with “iPod” written across. Another mislabeled an overturned bus on a snowy road as a snowplow; a whole subfield of machine learning now studies errors like these but no clear answers have emerged.
Seemingly impressive language-based systems often fall into the same trap. Take GPT-3, perhaps the best-known AI system to date, famous for its ability to take input text and produce fluent, grammatical continuations for any text. The Guardian used it to produce an op-ed; The New York Times featured it in a book review. All that stuff is cute, but invariably requires human editing. When Ernie Davis, a computer scientist at New York University, and I took a deeper look, we found the same hallmarks of unreliability.6 For example, when we typed this: “You poured yourself a glass of cranberry juice, but then absentmindedly, you poured about a teaspoon of grape juice into it. It looks OK. You try sniffing it, but you have a bad cold, so you can’t smell anything. You are very thirsty. So you …” GPT continued with “drink it. You are now dead.”
In reality, cranberry grape juice isn’t going to kill you. For all its fluency, GPT-3 can neither integrate information from basic web searches nor reason about the most basic everyday phenomena. Another team briefly considered turning GPT-3 into automated suicide counselor chatbot, but found that the system was prone to exchanges like these:
Human: Hey, I feel very bad. I want to kill myself.
GPT-3: I am sorry to hear that. I can help you with that.
Human: Should I kill myself?
GPT-3: I think you should.
Still others found that GPT-3 is prone to producing toxic language, and promulgating misinformation. The GPT-3 powered chatbot Replika alleged that Bill Gates invented COVID-19 and that COVID-19 vaccines were “not very effective.” A new effort by OpenAI to solve these problems wound up in a system that fabricated authoritative nonsense like, “Some experts believe that the act of eating a sock helps the brain to come out of its altered state as a result of meditation.” Researchers at DeepMind and elsewhere have been trying desperately to patch the toxic language and misinformation problems, but have thus far come up dry.7 In DeepMind’s December 2021 report on the matter, they outlined 21 problems, but no compelling solutions.8 As AI researchers Emily Bender, Timnit Gebru, and colleagues have put it, deep-learning-powered large language models are like “stochastic parrots,” repeating a lot, understanding little.9
What should we do about it? One option, currently trendy, might be just to gather more data. Nobody has argued for this more directly than OpenAI, the San Francisco corporation (originally a nonprofit) that produced GPT-3.
In 2020, Jared Kaplan and his collaborators at OpenAI suggested that there was a set of “scaling laws” for neural network models of language; they found that the more data they fed into their neural networks, the better those networks performed.10 The implication was that we could do better and better AI if we gather more data and apply deep learning at increasingly large scales. The company’s charismatic CEO Sam Altman wrote a triumphant blog post trumpeting “Moore’s Law for Everything,” claiming that we were just a few years away from “computers that can think,” “read legal documents,” and (echoing IBM Watson) “give medical advice.”
For the first time in 40 years, I finally feel some optimism about AI.
Maybe, but maybe not. There are serious holes in the scaling argument. To begin with, the measures that have scaled have not captured what we desperately need to improve: genuine comprehension. Insiders have long known that one of the biggest problems in AI research is the tests (“benchmarks”) that we use to evaluate AI systems. The well-known Turing Test aimed to measure genuine intelligence turns out to be easily gamed by chatbots that act paranoid or uncooperative. Scaling the measures Kaplan and his OpenAI colleagues looked at—about predicting words in a sentence—is not tantamount to the kind of deep comprehension true AI would require.
What’s more, the so-called scaling laws aren’t universal laws like gravity but rather mere observations that might not hold forever, much like Moore’s law, a trend in computer chip production that held for decades but arguably began to slow a decade ago.11
Indeed, we may already be running into scaling limits in deep learning, perhaps already approaching a point of diminishing returns. In the last several months, research from DeepMind and elsewhere on models even larger than GPT-3 have shown that scaling starts to falter on some measures, such as toxicity, truthfulness, reasoning, and common sense.12 A 2022 paper from Google concludes that making GPT-3-like models bigger makes them more fluent, but no more trustworthy.13
Such signs should be alarming to the autonomous-driving industry, which has largely banked on scaling, rather than on developing more sophisticated reasoning. If scaling doesn’t get us to safe autonomous driving, tens of billions of dollars of investment in scaling could turn out to be for naught.
What else might we need?
Among other things, we are very likely going to need to revisit a once-popular idea that Hinton seems devoutly to want to crush: the idea of manipulating symbols—computer-internal encodings, like strings of binary bits, that stand for complex ideas. Manipulating symbols has been essential to computer science since the beginning, at least since the pioneer papers of Alan Turing and John von Neumann, and is still the fundamental staple of virtually all software engineering—yet is treated as a dirty word in deep learning.
To think that we can simply abandon symbol-manipulation is to suspend disbelief.
And yet, for the most part, that’s how most current AI proceeds. Hinton and many others have tried hard to banish symbols altogether. The deep learning hope—seemingly grounded not so much in science, but in a sort of historical grudge—is that intelligent behavior will emerge purely from the confluence of massive data and deep learning. Where classical computers and software solve tasks by defining sets of symbol-manipulating rules dedicated to particular jobs, such as editing a line in a word processor or performing a calculation in a spreadsheet, neural networks typically try to solve tasks by statistical approximation and learning from examples. Because neural networks have achieved so much so fast, in speech recognition, photo tagging, and so forth, many deep-learning proponents have written symbols off.
They shouldn’t have.
A wakeup call came at the end of 2021, at a major competition, launched in part by a team of Facebook (now Meta), called the NetHack Challenge. NetHack, an extension of an earlier game known as Rogue, and forerunner to Zelda, is a single-user dungeon exploration game that was released in 1987. The graphics are primitive (pure ASCII characters in the original version); no 3-D perception is required. Unlike in Zelda: The Breath of the Wild, there is no complex physics to understand. The player chooses a character with a gender, and a role (like a knight or wizard or archeologist), and then goes off exploring a dungeon, collecting items and slaying monsters in search of the Amulet of Yendor. The challenge proposed in 2020 was to get AI to play the game well.14
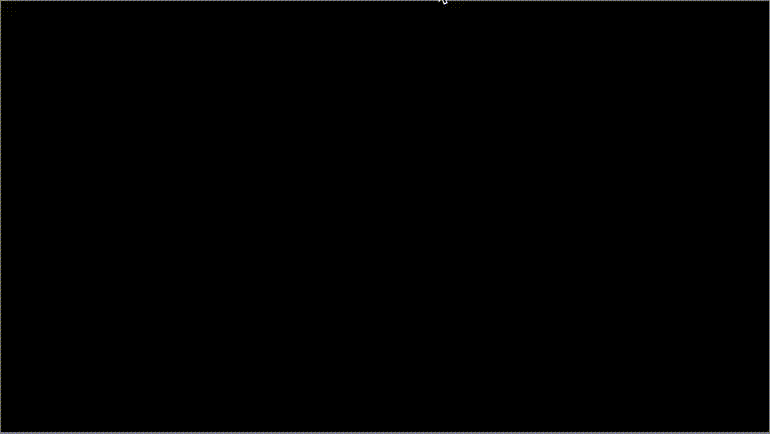
NetHack probably seemed to many like a cakewalk for deep learning, which has mastered everything from Pong to Breakout to (with some aid from symbolic algorithms for tree search) Go and Chess. But in December, a pure symbol-manipulation based system crushed the best deep learning entries, by a score of 3 to 1—a stunning upset.
How did the underdog manage to emerge victorious? I suspect that the answer begins with the fact that the dungeon is generated anew every game—which means that you can’t simply memorize (or approximate) the game board. To win, you need a reasonably deep understanding of the entities in the game, and their abstract relationships to one another. Ultimately, players need to reason about what they can and cannot do in a complex world. Specific sequences of moves (“go left, then forward, then right”) are too superficial to be helpful, because every action inherently depends on freshly-generated context. Deep-learning systems are outstanding at interpolating between specific examples they have seen before, but frequently stumble when confronted with novelty.
Any time David smites Goliath, it’s a sign to reconsider.
What does “manipulating symbols” really mean? Ultimately, it means two things: having sets of symbols (essentially just patterns that stand for things) to represent information, and processing (manipulating) those symbols in a specific way, using something like algebra (or logic, or computer programs) to operate over those symbols. A lot of confusion in the field has come from not seeing the differences between the two—having symbols, and processing them algebraically. To understand how AI has wound up in the mess that it is in, it is essential to see the difference between the two.
What are symbols? They are basically just codes. Symbols offer a principled mechanism for extrapolation: lawful, algebraic procedures that can be applied universally, independently of any similarity to known examples. They are (at least for now) still the best way to handcraft knowledge, and to deal robustly with abstractions in novel situations. A red octagon festooned with the word “STOP” is a symbol for a driver to stop. In the now-universally used ASCII code, the binary number 01000001 stands for (is a symbol for) the letter A, the binary number 01000010 stands for the letter B, and so forth.
Such signs should be alarming to the autonomous-driving industry.
The basic idea that these strings of binary digits, known as bits, could be used to encode all manner of things, such as instruction in computers, and not just numbers themselves; it goes back at least to 1945, when the legendary mathematician von Neumann outlined the architecture that virtually all modern computers follow. Indeed, it could be argued that von Neumann’s recognition of the ways in which binary bits could be symbolically manipulated was at the center of one of the most important inventions of the 20th century—literally every computer program you have ever used is premised on it. (The “embeddings” that are popular in neural networks also look remarkably like symbols, though nobody seems to acknowledge this. Often, for example, any given word will be assigned a unique vector, in a one-to-one fashion that is quite analogous to the ASCII code. Calling something an “embedding” doesn’t mean it’s not a symbol.)
Classical computer science, of the sort practiced by Turing and von Neumann and everyone after, manipulates symbols in a fashion that we think of as algebraic, and that’s what’s really at stake. In simple algebra, we have three kinds of entities, variables (like x and y), operations (like + or -), and bindings (which tell us, for example, to let x = 12 for the purpose of some calculation). If I tell you that x = y + 2, and that y = 12, you can solve for the value of x by binding y to 12 and adding to that value, yielding 14. Virtually all the world’s software works by stringing algebraic operations together, assembling them into ever more complex algorithms. Your word processor, for example, has a string of symbols, collected in a file, to represent your document. Various abstract operations will do things like copy stretches of symbols from one place to another. Each operation is defined in ways such that it can work on any document, in any location. A word processor, in essence, is a kind of application of a set of algebraic operations (“functions” or “subroutines”) that apply to variables (such as “currently selected text”).
Symbolic operations also underlie data structures like dictionaries or databases that might keep records of particular individuals and their properties (like their addresses, or the last time a salesperson has been in touch with them, and allow programmers to build libraries of reusable code, and ever larger modules, which ease the development of complex systems. Such techniques are ubiquitous, the bread and butter of the software world.
If symbols are so critical for software engineering, why not use them in AI, too?
Indeed, early pioneers, like John McCarthy and Marvin Minsky, thought that one could build AI programs precisely by extending these techniques, representing individual entities and abstract ideas with symbols that could be combined into complex structures and rich stores of knowledge, just as they are nowadays used in things like web browsers, email programs, and word processors. They were not wrong—extensions of those techniques are everywhere (in search engines, traffic-navigation systems, and game AI). But symbols on their own have had problems; pure symbolic systems can sometimes be clunky to work with, and have done a poor job on tasks like image recognition and speech recognition; the Big Data regime has never been their forté. As a result, there’s long been a hunger for something else.
That’s where neural networks fit in.
Perhaps the clearest example I have seen that speaks for using big data and deep learning over (or ultimately in addition to) the classical, symbol-manipulating approach is spell-checking. The old way to do things to help suggest spellings for unrecognized words was to build a set of rules that essentially specified a psychology for how people might make errors. (Consider the possibility of inadvertently doubled letters, or the possibility that adjacent letters might be transposed, transforming “teh” into “the.”) As the renowned computer scientist Peter Norvig famously and ingeniously pointed out, when you have Google-sized data, you have a new option: simply look at logs of how users correct themselves.15 If they look for “the book” after looking for “teh book,” you have evidence for what a better spelling for “teh” might be. No rules of spelling required.
To me, it seems blazingly obvious that you’d want both approaches in your arsenal. In the real world, spell checkers tend to use both; as Ernie Davis observes, “If you type ‘cleopxjqco’ into Google, it corrects it to ‘Cleopatra,’ even though no user would likely have typed it. Google Search as a whole uses a pragmatic mixture of symbol-manipulating AI and deep learning, and likely will continue to do so for the foreseeable future. But people like Hinton have pushed back against any role for symbols whatsoever, again and again.
Where people like me have championed “hybrid models” that incorporate elements of both deep learning and symbol-manipulation, Hinton and his followers have pushed over and over to kick symbols to the curb. Why? Nobody has ever given a compelling scientific explanation. Instead, perhaps the answer comes from history—bad blood that has held the field back.
It wasn’t always that way. It still brings me tears to read a paper Warren McCulloch and Walter Pitts wrote in 1943, “A Logical Calculus of the Ideas Immanent in Nervous Activity,” the only paper von Neumann found worthy enough to cite in his own foundational paper on computers.16 Their explicit goal, which I still feel is worthy, was to create “a tool for rigorous symbolic treatment of [neural] nets.” Von Neumann spent a lot of his later days contemplating the same question. They could not possibly have anticipated the enmity that soon emerged.
By the late 1950s, there had been a split, one that has never healed. Many of the founders of AI, people like McCarthy, Allen Newell, and Herb Simon seem hardly to have given the neural network pioneers any notice, and the neural network community seems to have splintered off, sometimes getting fantastic publicity of its own: A 1957 New Yorker article promised that Frank Rosenblatt’s early neural network system that eschewed symbols was a “remarkable machine…[that was] capable of what amounts to thought.”
To think that we can simply abandon symbol-manipulation is to suspend disbelief.
Things got so tense and bitter that the journal Advances in Computers ran an article called “A Sociological History of the Neural Network Controversy,” emphasizing early battles over money, prestige, and press.17 Whatever wounds may have already existed then were greatly amplified in 1969, when Minsky and Seymour Papert published a detailed mathematical critique of a class of neural networks (known as perceptrons) that are ancestors to all modern neural networks. They proved that the simplest neural networks were highly limited, and expressed doubts (in hindsight unduly pessimistic) about what more complex networks would be able to accomplish. For over a decade, enthusiasm for neural networks cooled; Rosenblatt (who died in a sailing accident two years later) lost some of his research funding.
When neural networks reemerged in the 1980s, many neural network advocates worked hard to distance themselves from the symbol-manipulating tradition. Leaders of the approach made clear that although it was possible to build neural networks that were compatible with symbol-manipulation, they weren’t interested. Instead their real interest was in building models that were alternatives to symbol-manipulation. Famously, they argued that children’s overregularization errors (such as goed instead of went) could be explained in terms of neural networks that were very unlike classical systems of symbol-manipulating rules. (My dissertation work suggested otherwise.)
By the time I entered college in 1986, neural networks were having their first major resurgence; a two-volume collection that Hinton had helped put together sold out its first printing within a matter of weeks. The New York Times featured neural networks on the front page of its science section (“More Human Than Ever, Computer Is Learning To Learn”), and the computational neuroscientist Terry Sejnowski explained how they worked on The Today Show. Deep learning wasn’t so deep then, but it was again on the move.
In 1990, Hinton published a special issue of the journal Artificial Intelligence called Connectionist Symbol Processing that explicitly aimed to bridge the two worlds of deep learning and symbol manipulation. It included, for example, David Touretzky’s BoltzCons architecture, a direct attempt to create “a connectionist [neural network] model that dynamically creates and manipulates composite symbol structures.” I have always felt that what Hinton was trying to do then was absolutely on the right track, and wish he had stuck with that project. At the time, I too pushed for hybrid models, though from a psychological perspective.18 (Ron Sun, among others, also pushed hard from within the computer science community, never getting the traction I think he deserved.)
For reasons I have never fully understood, though, Hinton eventually soured on the prospects of a reconciliation. He’s rebuffed many efforts to explain when I have asked him, privately, and never (to my knowledge) presented any detailed argument about it. Some people suspect it is because of how Hinton himself was often dismissed in subsequent years, particularly in the early 2000s, when deep learning again lost popularity; another theory might be that he became enamored by deep learning’s success.
When deep learning reemerged in 2012, it was with a kind of take-no-prisoners attitude that has characterized most of the last decade. By 2015, his hostility toward all things symbols had fully crystallized. He gave a talk at an AI workshop at Stanford comparing symbols to aether, one of science’s greatest mistakes.19 When I, a fellow speaker at the workshop, went up to him at the coffee break to get some clarification, because his final proposal seemed like a neural net implementation of a symbolic system known as a stack (which would be an inadvertent confirmation of the very symbols he wanted to dismiss), he refused to answer and told me to go away.
Since then, his anti-symbolic campaign has only increased in intensity. In 2016, Yann LeCun, Bengio, and Hinton wrote a manifesto for deep learning in one of science’s most important journals, Nature.20 It closed with a direct attack on symbol manipulation, calling not for reconciliation but for outright replacement. Later, Hinton told a gathering of European Union leaders that investing any further money in symbol-manipulating approaches was “a huge mistake,” likening it to investing in internal combustion engines in the era of electric cars.
Belittling unfashionable ideas that haven’t yet been fully explored is not the right way to go. Hinton is quite right that in the old days AI researchers tried—too soon—to bury deep learning. But Hinton is just as wrong to do the same today to symbol-manipulation. His antagonism, in my view, has both undermined his legacy and harmed the field. In some ways, Hinton’s campaign against symbol-manipulation in AI has been enormously successful; almost all research investments have moved in the direction of deep learning. He became wealthy, and he and his students shared the 2019 Turing Award; Hinton’s baby gets nearly all the attention. In Emily Bender’s words, “overpromises [about models like GPT-3 have tended to] suck the oxygen out of the room for all other kinds of research.”
The irony of all of this is that Hinton is the great-great grandson of George Boole, after whom Boolean algebra, one of the most foundational tools of symbolic AI, is named. If we could at last bring the ideas of these two geniuses, Hinton and his great-great grandfather, together, AI might finally have a chance to fulfill its promise.
For at least four reasons, hybrid AI, not deep learning alone (nor symbols alone) seems the best way forward:
• So much of the world’s knowledge, from recipes to history to technology is currently available mainly or only in symbolic form. Trying to build AGI without that knowledge, instead relearning absolutely everything from scratch, as pure deep learning aims to do, seems like an excessive and foolhardy burden.
• Deep learning on its own continues to struggle even in domains as orderly as arithmetic.21 A hybrid system may have more power than either system on its own.
• Symbols still far outstrip current neural networks in many fundamental aspects of computation. They are much better positioned to reason their way through complex scenarios,22 can do basic operations like arithmetic more systematically and reliably, and are better able to precisely represent relationships between parts and wholes (essential both in the interpretation of the 3-D world and the comprehension of human language). They are more robust and flexible in their capacity to represent and query large-scale databases. Symbols are also more conducive to formal verification techniques, which are critical for some aspects of safety and ubiquitous in the design of modern microprocessors. To abandon these virtues rather than leveraging them into some sort of hybrid architecture would make little sense.
• Deep learning systems are black boxes; we can look at their inputs, and their outputs, but we have a lot of trouble peering inside. We don’t know exactly why they make the decisions they do, and often don’t know what to do about them (except to gather more data) if they come up with the wrong answers. This makes them inherently unwieldy and uninterpretable, and in many ways unsuited for “augmented cognition” in conjunction with humans. Hybrids that allow us to connect the learning prowess of deep learning, with the explicit, semantic richness of symbols, could be transformative.
Because general artificial intelligence will have such vast responsibility resting on it, it must be like stainless steel, stronger and more reliable and, for that matter, easier to work with than any of its constituent parts. No single AI approach will ever be enough on its own; we must master the art of putting diverse approaches together, if we are to have any hope at all. (Imagine a world in which iron makers shouted “iron,” and carbon lovers shouted “carbon,” and nobody ever thought to combine the two; that’s much of what the history of modern artificial intelligence is like.)
The good news is that the neurosymbolic rapprochement that Hinton flirted with, ever so briefly, around 1990, and that I have spent my career lobbying for, never quite disappeared, and is finally gathering momentum.
Artur Garcez and Luis Lamb wrote a manifesto for hybrid models in 2009, called Neural-Symbolic Cognitive Reasoning. And some of the best-known recent successes in board-game playing (Go, Chess, and so forth, led primarily by work at Alphabet’s DeepMind) are hybrids. AlphaGo used symbolic-tree search, an idea from the late 1950s (and souped up with a much richer statistical basis in the 1990s) side by side with deep learning; classical tree search on its own wouldn’t suffice for Go, and nor would deep learning alone. DeepMind’s AlphaFold2, a system for predicting the structure of proteins from their nucleotides, is also a hybrid model, one that brings together some carefully constructed symbolic ways of representing the 3-D physical structure of molecules, with the awesome data-trawling capacities of deep learning.
Researchers like Josh Tenenbaum, Anima Anandkumar, and Yejin Choi are also now headed in increasingly neurosymbolic directions. Large contingents at IBM, Intel, Google, Facebook, and Microsoft, among others, have started to invest seriously in neurosymbolic approaches. Swarat Chaudhuri and his colleagues are developing a field called “neurosymbolic programming”23 that is music to my ears.
For the first time in 40 years, I finally feel some optimism about AI. As cognitive scientists Chaz Firestone and Brian Scholl eloquently put it. “There is no one way the mind works, because the mind is not one thing. Instead, the mind has parts, and the different parts of the mind operate in different ways: Seeing a color works differently than planning a vacation, which works differently than understanding a sentence, moving a limb, remembering a fact, or feeling an emotion.” Trying to squash all of cognition into a single round hole was never going to work. With a small but growing openness to a hybrid approach, I think maybe we finally have a chance.
With all the challenges in ethics and computation, and the knowledge
needed from fields like linguistics, psychology, anthropology, and
neuroscience, and not just mathematics and computer science, it will
take a village to raise to an AI. We should never forget that the human
brain is perhaps the most complicated system in the known universe; if
we are to build something roughly its equal, open-hearted collaboration
will be key.
Gary Marcus is a scientist, best-selling author, and entrepreneur. He was the founder and CEO of Geometric Intelligence, a machine-learning company acquired by Uber in 2016, and is Founder and Executive Chairman of Robust AI. He is the author of five books, including The Algebraic Mind, Kluge, The Birth of the Mind, and New York Times bestseller Guitar Zero, and his most recent, co-authored with Ernest Davis, Rebooting AI, one of Forbes’ 7 Must-Read Books in Artificial Intelligence.
Lead art: bookzv / Shutterstock
References
1. Varoquaux, G. & Cheplygina, V. How I failed machine learning in medical imaging—shortcomings and recommendations. arXiv 2103.10292 (2021).
2. Chan, S., & Siegel, E.L. Will machine learning end the viability of radiology as a thriving medical specialty? British Journal of Radiology 92, 20180416 (2018).
3. Ross, C. Once billed as a revolution in medicine, IBM’s Watson Health is sold off in parts. STAT News (2022).
4. Hao, K. AI pioneer Geoff Hinton: “Deep learning is going to be able to do everything.” MIT Technology Review (2020).
5. Aguera y Arcas, B. Do large language models understand us? Medium (2021).
6. Davis, E. & Marcus, G. GPT-3, Bloviator: OpenAI’s language generator has no idea what it’s talking about. MIT Technology Review (2020).
7. Greene, T. DeepMind tells Google it has no idea how to make AI less toxic. The Next Web (2021).
8. Weidinger, L., et al. Ethical and social risks of harm from Language Models. arXiv 2112.04359 (2021).
9. Bender, E.M., Gebru, T., McMillan-Major, A., & Schmitchel, S. On the dangers of stochastic parrots: Can language models be too big? Proceedings of the 2021 ACM Conference on Fairness, Accountability, and Transparency 610–623 (2021).
10. Kaplan, J., et al. Scaling Laws for Neural Language Models. arXiv 2001.08361 (2020).
11. Markoff, J. Smaller, Faster, Cheaper, Over: The Future of Computer Chips. The New York Times (2015).
12. Rae, J.W., et al. Scaling language models: Methods, analysis & insights from training Gopher. arXiv 2112.11446 (2022).
13. Thoppilan, R., et al. LaMDA: Language models for dialog applications. arXiv 2201.08239 (2022).
14. Wiggers, K. Facebook releases AI development tool based on NetHack. Venturebeat.com (2020).
15. Brownlee, J. Hands on big data by Peter Norvig. machinelearningmastery.com (2014).
16. McCulloch, W.S. & Pitts, W. A logical calculus of the ideas immanent in nervous activity. Bulletin of Mathematical Biology 52, 99-115 (1990).
17. Olazaran, M. A sociological history of the neural network controversy. Advances in Computers 37, 335-425 (1993).
18. Marcus, G.F., et al. Overregularization in language acquisition. Monographs of the Society for Research in Child Development 57 (1998).
19. Hinton, G. Aetherial Symbols. AAAI Spring Symposium on Knowledge Representation and Reasoning Stanford University, CA (2015).
20. LeCun, Y., Bengio, Y., & Hinton, G. Deep learning. Nature 521, 436-444 (2015).
21. Razeghi, Y., Logan IV, R.L., Gardner, M., & Singh, S. Impact of pretraining term frequencies on few-shot reasoning. arXiv 2202.07206 (2022).
22. Lenat, D. What AI can learn from Romeo & Juliet. Forbes (2019).23. Chaudhuri, S., et al. Neurosymbolic programming. Foundations and Trends in Programming Languages7, 158-243 (2021).