
Collective intelligence (CI) is shared or group intelligence (GI) that emerges from the collaboration, collective efforts, and competition of many individuals and appears in consensus decision making. The term appears in sociobiology, political science and in context of mass peer review and crowdsourcing applications. It may involve consensus, social capital and formalisms such as voting systems, social media and other means of quantifying mass activity. Collective IQ is a measure of collective intelligence, although it is often used interchangeably with the term collective intelligence. Collective intelligence has also been attributed to bacteria and animals.
It can be understood as an emergent property from the synergies among:
- data-information-knowledge
- software-hardware
- individuals (those with new insights as well as recognized authorities) that continually learn from feedback to produce just-in-time knowledge for better decisions than these three elements acting alone
Or it can be more narrowly understood as an emergent property between people and ways of processing information. This notion of collective intelligence is referred to as "symbiotic intelligence" by Norman Lee Johnson. The concept is used in sociology, business, computer science and mass communications: it also appears in science fiction. Pierre Lévy defines collective intelligence as, "It is a form of universally distributed intelligence, constantly enhanced, coordinated in real time, and resulting in the effective mobilization of skills. I'll add the following indispensable characteristic to this definition: The basis and goal of collective intelligence is mutual recognition and enrichment of individuals rather than the cult of fetishized or hypostatized communities." According to researchers Pierre Lévy and Derrick de Kerckhove, it refers to capacity of networked ICTs (Information communication technologies) to enhance the collective pool of social knowledge by simultaneously expanding the extent of human interactions. A broader definition was provided by Geoff Mulgan in a series of lectures and reports from 2006 onwards and in the book Big Mind which proposed a framework for analysing any thinking system, including both human and machine intelligence, in terms of functional elements (observation, prediction, creativity, judgement etc.), learning loops and forms of organisation. The aim was to provide a way to diagnose, and improve, the collective intelligence of a city, business, NGO or parliament.
Collective intelligence strongly contributes to the shift of knowledge and power from the individual to the collective. According to Eric S. Raymond in 1998 and JC Herz in 2005, open-source intelligence will eventually generate superior outcomes to knowledge generated by proprietary software developed within corporations. Media theorist Henry Jenkins sees collective intelligence as an 'alternative source of media power', related to convergence culture. He draws attention to education and the way people are learning to participate in knowledge cultures outside formal learning settings. Henry Jenkins criticizes schools which promote 'autonomous problem solvers and self-contained learners' while remaining hostile to learning through the means of collective intelligence. Both Pierre Lévy and Henry Jenkins support the claim that collective intelligence is important for democratization, as it is interlinked with knowledge-based culture and sustained by collective idea sharing, and thus contributes to a better understanding of diverse society.
Similar to the g factor (g) for general individual intelligence, a new scientific understanding of collective intelligence aims to extract a general collective intelligence factor c factor for groups indicating a group's ability to perform a wide range of tasks. Definition, operationalization and statistical methods are derived from g. Similarly as g is highly interrelated with the concept of IQ, this measurement of collective intelligence can be interpreted as intelligence quotient for groups (Group-IQ) even though the score is not a quotient per se. Causes for c and predictive validity are investigated as well.
Writers who have influenced the idea of collective intelligence include Francis Galton, Douglas Hofstadter (1979), Peter Russell (1983), Tom Atlee (1993), Pierre Lévy (1994), Howard Bloom (1995), Francis Heylighen (1995), Douglas Engelbart, Louis Rosenberg, Cliff Joslyn, Ron Dembo, Gottfried Mayer-Kress (2003), and Geoff Mulgan.
History

The concept (although not so named) originated in 1785 with the Marquis de Condorcet, whose "jury theorem" states that if each member of a voting group is more likely than not to make a correct decision, the probability that the highest vote of the group is the correct decision increases with the number of members of the group. Many theorists have interpreted Aristotle's statement in the Politics that "a feast to which many contribute is better than a dinner provided out of a single purse" to mean that just as many may bring different dishes to the table, so in a deliberation many may contribute different pieces of information to generate a better decision. Recent scholarship, however, suggests that this was probably not what Aristotle meant but is a modern interpretation based on what we now know about team intelligence.
A precursor of the concept is found in entomologist William Morton Wheeler's observation in 1910 that seemingly independent individuals can cooperate so closely as to become indistinguishable from a single organism. Wheeler saw this collaborative process at work in ants that acted like the cells of a single beast he called a superorganism.
In 1912 Émile Durkheim identified society as the sole source of human logical thought. He argued in "The Elementary Forms of Religious Life" that society constitutes a higher intelligence because it transcends the individual over space and time. Other antecedents are Vladimir Vernadsky and Pierre Teilhard de Chardin's concept of "noosphere" and H. G. Wells's concept of "world brain". Peter Russell, Elisabet Sahtouris, and Barbara Marx Hubbard (originator of the term "conscious evolution") are inspired by the visions of a noosphere – a transcendent, rapidly evolving collective intelligence – an informational cortex of the planet. The notion has more recently been examined by the philosopher Pierre Lévy. In a 1962 research report, Douglas Engelbart linked collective intelligence to organizational effectiveness, and predicted that pro-actively 'augmenting human intellect' would yield a multiplier effect in group problem solving: "Three people working together in this augmented mode [would] seem to be more than three times as effective in solving a complex problem as is one augmented person working alone". In 1994, he coined the term 'collective IQ' as a measure of collective intelligence, to focus attention on the opportunity to significantly raise collective IQ in business and society.
The idea of collective intelligence also forms the framework for contemporary democratic theories often referred to as epistemic democracy. Epistemic democratic theories refer to the capacity of the populace, either through deliberation or aggregation of knowledge, to track the truth and relies on mechanisms to synthesize and apply collective intelligence.
Collective intelligence was introduced into the machine learning community in the late 20th century, and matured into a broader consideration of how to design "collectives" of self-interested adaptive agents to meet a system-wide goal. This was related to single-agent work on "reward shaping" and has been taken forward by numerous researchers in the game theory and engineering communities.
Dimensions

Howard Bloom has discussed mass behavior – collective behavior from the level of quarks to the level of bacterial, plant, animal, and human societies. He stresses the biological adaptations that have turned most of this earth's living beings into components of what he calls "a learning machine". In 1986 Bloom combined the concepts of apoptosis, parallel distributed processing, group selection, and the superorganism to produce a theory of how collective intelligence works. Later he showed how the collective intelligences of competing bacterial colonies and human societies can be explained in terms of computer-generated "complex adaptive systems" and the "genetic algorithms", concepts pioneered by John Holland.
Bloom traced the evolution of collective intelligence to our bacterial ancestors 1 billion years ago and demonstrated how a multi-species intelligence has worked since the beginning of life. Ant societies exhibit more intelligence, in terms of technology, than any other animal except for humans and co-operate in keeping livestock, for example aphids for "milking". Leaf cutters care for fungi and carry leaves to feed the fungi.
David Skrbina cites the concept of a 'group mind' as being derived from Plato's concept of panpsychism (that mind or consciousness is omnipresent and exists in all matter). He develops the concept of a 'group mind' as articulated by Thomas Hobbes in Leviathan and Fechner's arguments for a collective consciousness of mankind. He cites Durkheim as the most notable advocate of a "collective consciousness" and Teilhard de Chardin as a thinker who has developed the philosophical implications of the group mind.
Tom Atlee focuses primarily on humans and on work to upgrade what Howard Bloom calls "the group IQ". Atlee feels that collective intelligence can be encouraged "to overcome 'groupthink' and individual cognitive bias in order to allow a collective to cooperate on one process – while achieving enhanced intellectual performance." George Pór defined the collective intelligence phenomenon as "the capacity of human communities to evolve towards higher order complexity and harmony, through such innovation mechanisms as differentiation and integration, competition and collaboration." Atlee and Pór state that "collective intelligence also involves achieving a single focus of attention and standard of metrics which provide an appropriate threshold of action". Their approach is rooted in scientific community metaphor.
The term group intelligence is sometimes used interchangeably with the term collective intelligence. Anita Woolley presents Collective intelligence as a measure of group intelligence and group creativity. The idea is that a measure of collective intelligence covers a broad range of features of the group, mainly group composition and group interaction. The features of composition that lead to increased levels of collective intelligence in groups include criteria such as higher numbers of women in the group as well as increased diversity of the group.
Atlee and Pór suggest that the field of collective intelligence should primarily be seen as a human enterprise in which mind-sets, a willingness to share and an openness to the value of distributed intelligence for the common good are paramount, though group theory and artificial intelligence have something to offer. Individuals who respect collective intelligence are confident of their own abilities and recognize that the whole is indeed greater than the sum of any individual parts. Maximizing collective intelligence relies on the ability of an organization to accept and develop "The Golden Suggestion", which is any potentially useful input from any member. Groupthink often hampers collective intelligence by limiting input to a select few individuals or filtering potential Golden Suggestions without fully developing them to implementation.
Robert David Steele Vivas in The New Craft of Intelligence portrayed all citizens as "intelligence minutemen", drawing only on legal and ethical sources of information, able to create a "public intelligence" that keeps public officials and corporate managers honest, turning the concept of "national intelligence" (previously concerned about spies and secrecy) on its head.

According to Don Tapscott and Anthony D. Williams, collective intelligence is mass collaboration. In order for this concept to happen, four principles need to exist:
- Openness – Sharing ideas and intellectual property: though these resources provide the edge over competitors more benefits accrue from allowing others to share ideas and gain significant improvement and scrutiny through collaboration.
- Peering – Horizontal organization as with the 'opening up' of the Linux program where users are free to modify and develop it provided that they make it available for others. Peering succeeds because it encourages self-organization – a style of production that works more effectively than hierarchical management for certain tasks.
- Sharing – Companies have started to share some ideas while maintaining some degree of control over others, like potential and critical patent rights. Limiting all intellectual property shuts out opportunities, while sharing some expands markets and brings out products faster.
- Acting globally – The advancement in communication technology has prompted the rise of global companies at low overhead costs. The internet is widespread, therefore a globally integrated company has no geographical boundaries and may access new markets, ideas and technology.
Collective intelligence factor c

A new scientific understanding of collective intelligence defines it as a group's general ability to perform a wide range of tasks. Definition, operationalization and statistical methods are similar to the psychometric approach of general individual intelligence. Hereby, an individual's performance on a given set of cognitive tasks is used to measure general cognitive ability indicated by the general intelligence factor g proposed by English psychologist Charles Spearman and extracted via factor analysis. In the same vein as g serves to display between-individual performance differences on cognitive tasks, collective intelligence research aims to find a parallel intelligence factor for groups 'c factor' (also called 'collective intelligence factor' (CI)) displaying between-group differences on task performance. The collective intelligence score then is used to predict how this same group will perform on any other similar task in the future. Yet tasks, hereby, refer to mental or intellectual tasks performed by small groups even though the concept is hoped to be transferable to other performances and any groups or crowds reaching from families to companies and even whole cities. Since individuals' g factor scores are highly correlated with full-scale IQ scores, which are in turn regarded as good estimates of g, this measurement of collective intelligence can also be seen as an intelligence indicator or quotient respectively for a group (Group-IQ) parallel to an individual's intelligence quotient (IQ) even though the score is not a quotient per se.
Mathematically, c and g are both variables summarizing positive correlations among different tasks supposing that performance on one task is comparable with performance on other similar tasks. c thus is a source of variance among groups and can only be considered as a group's standing on the c factor compared to other groups in a given relevant population. The concept is in contrast to competing hypotheses including other correlational structures to explain group intelligence, such as a composition out of several equally important but independent factors as found in individual personality research.
Besides, this scientific idea also aims to explore the causes affecting collective intelligence, such as group size, collaboration tools or group members' interpersonal skills. The MIT Center for Collective Intelligence, for instance, announced the detection of The Genome of Collective Intelligence as one of its main goals aiming to develop a "taxonomy of organizational building blocks, or genes, that can be combined and recombined to harness the intelligence of crowds".
Causes
Individual intelligence is shown to be genetically and environmentally influenced. Analogously, collective intelligence research aims to explore reasons why certain groups perform more intelligently than other groups given that c is just moderately correlated with the intelligence of individual group members. According to Woolley et al.'s results, neither team cohesion nor motivation or satisfaction is correlated with c. However, they claim that three factors were found as significant correlates: the variance in the number of speaking turns, group members' average social sensitivity and the proportion of females. All three had similar predictive power for c, but only social sensitivity was statistically significant (b=0.33, P=0.05).
The number speaking turns indicates that "groups where a few people dominated the conversation were less collectively intelligent than those with a more equal distribution of conversational turn-taking". Hence, providing multiple team members the chance to speak up made a group more intelligent.
Group members' social sensitivity was measured via the Reading the Mind in the Eyes Test (RME) and correlated .26 with c. Hereby, participants are asked to detect thinking or feeling expressed in other peoples' eyes presented on pictures and assessed in a multiple choice format. The test aims to measure peoples' theory of mind (ToM), also called 'mentalizing' or 'mind reading', which refers to the ability to attribute mental states, such as beliefs, desires or intents, to other people and in how far people understand that others have beliefs, desires, intentions or perspectives different from their own ones. RME is a ToM test for adults that shows sufficient test-retest reliability and constantly differentiates control groups from individuals with functional autism or Asperger Syndrome. It is one of the most widely accepted and well-validated tests for ToM within adults. ToM can be regarded as an associated subset of skills and abilities within the broader concept of emotional intelligence.
The proportion of females as a predictor of c was largely mediated by social sensitivity (Sobel z = 1.93, P= 0.03) which is in vein with previous research showing that women score higher on social sensitivity tests. While a mediation, statistically speaking, clarifies the mechanism underlying the relationship between a dependent and an independent variable, Wolley agreed in an interview with the Harvard Business Review that these findings are saying that groups of women are smarter than groups of men. However, she relativizes this stating that the actual important thing is the high social sensitivity of group members.
It is theorized that the collective intelligence factor c is an emergent property resulting from bottom-up as well as top-down processes. Hereby, bottom-up processes cover aggregated group-member characteristics. Top-down processes cover group structures and norms that influence a group's way of collaborating and coordinating.
Processes

Top-down processes
Top-down processes cover group interaction, such as structures, processes, and norms. An example of such top-down processes is conversational turn-taking. Research further suggest that collectively intelligent groups communicate more in general as well as more equally; same applies for participation and is shown for face-to-face as well as online groups communicating only via writing.
Bottom-up processes
Bottom-up processes include group composition, namely the characteristics of group members which are aggregated to the team level. An example of such bottom-up processes is the average social sensitivity or the average and maximum intelligence scores of group members. Furthermore, collective intelligence was found to be related to a group's cognitive diversity including thinking styles and perspectives. Groups that are moderately diverse in cognitive style have higher collective intelligence than those who are very similar in cognitive style or very different. Consequently, groups where members are too similar to each other lack the variety of perspectives and skills needed to perform well. On the other hand, groups whose members are too different seem to have difficulties to communicate and coordinate effectively.
Serial vs Parallel processes
For most of human history, collective intelligence was confined to small tribal groups in which opinions were aggregated through real-time parallel interactions among members. In modern times, mass communication, mass media, and networking technologies have enabled collective intelligence to span massive groups, distributed across continents and time-zones. To accommodate this shift in scale, collective intelligence in large-scale groups been dominated by serialized polling processes such as aggregating up-votes, likes, and ratings over time. While modern systems benefit from larger group size, the serialized process has been found to introduce substantial noise that distorts the collective output of the group. In one significant study of serialized collective intelligence, it was found that the first vote contributed to a serialized voting system can distort the final result by 34%.
To address the problems of serialized aggregation of input among large-scale groups, recent advancements collective intelligence have worked to replace serialized votes, polls, and markets, with parallel systems such as "human swarms" modeled after synchronous swarms in nature. Based on natural process of Swarm Intelligence, these artificial swarms of networked humans enable participants to work together in parallel to answer questions and make predictions as an emergent collective intelligence. In one high-profile example, a human swarm challenge by CBS Interactive to predict the Kentucky Derby. The swarm correctly predicted the first four horses, in order, defying 542–1 odds and turning a $20 bet into $10,800.
The value of parallel collective intelligence was demonstrated in medical applications by researchers at Stanford University School of Medicine and Unanimous AI in a set of published studies wherein groups of human doctors were connected by real-time swarming algorithms and tasked with diagnosing chest x-rays for the presence of pneumonia. When working together as "human swarms," the groups of experienced radiologists demonstrated a 33% reduction in diagnostic errors as compared to traditional methods.
Evidence
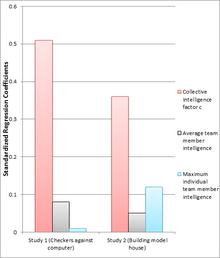
Woolley, Chabris, Pentland, Hashmi, & Malone (2010), the originators of this scientific understanding of collective intelligence, found a single statistical factor for collective intelligence in their research across 192 groups with people randomly recruited from the public. In Woolley et al.'s two initial studies, groups worked together on different tasks from the McGrath Task Circumplex, a well-established taxonomy of group tasks. Tasks were chosen from all four quadrants of the circumplex and included visual puzzles, brainstorming, making collective moral judgments, and negotiating over limited resources. The results in these tasks were taken to conduct a factor analysis. Both studies showed support for a general collective intelligence factor c underlying differences in group performance with an initial eigenvalue accounting for 43% (44% in study 2) of the variance, whereas the next factor accounted for only 18% (20%). That fits the range normally found in research regarding a general individual intelligence factor g typically accounting for 40% to 50% percent of between-individual performance differences on cognitive tests.
Afterwards, a more complex task was solved by each group to determine whether c factor scores predict performance on tasks beyond the original test. Criterion tasks were playing checkers (draughts) against a standardized computer in the first and a complex architectural design task in the second study. In a regression analysis using both individual intelligence of group members and c to predict performance on the criterion tasks, c had a significant effect, but average and maximum individual intelligence had not. While average (r=0.15, P=0.04) and maximum intelligence (r=0.19, P=0.008) of individual group members were moderately correlated with c, c was still a much better predictor of the criterion tasks. According to Woolley et al., this supports the existence of a collective intelligence factor c, because it demonstrates an effect over and beyond group members' individual intelligence and thus that c is more than just the aggregation of the individual IQs or the influence of the group member with the highest IQ.
Engel et al. (2014) replicated Woolley et al.'s findings applying an accelerated battery of tasks with a first factor in the factor analysis explaining 49% of the between-group variance in performance with the following factors explaining less than half of this amount. Moreover, they found a similar result for groups working together online communicating only via text and confirmed the role of female proportion and social sensitivity in causing collective intelligence in both cases. Similarly to Wolley et al., they also measured social sensitivity with the RME which is actually meant to measure people's ability to detect mental states in other peoples' eyes. The online collaborating participants, however, did neither know nor see each other at all. The authors conclude that scores on the RME must be related to a broader set of abilities of social reasoning than only drawing inferences from other people's eye expressions.
A collective intelligence factor c in the sense of Woolley et al. was further found in groups of MBA students working together over the course of a semester, in online gaming groups as well as in groups from different cultures and groups in different contexts in terms of short-term versus long-term groups. None of these investigations considered team members' individual intelligence scores as control variables.
Note as well that the field of collective intelligence research is quite young and published empirical evidence is relatively rare yet. However, various proposals and working papers are in progress or already completed but (supposedly) still in a scholarly peer reviewing publication process.
Predictive validity
Next to predicting a group's performance on more complex criterion tasks as shown in the original experiments, the collective intelligence factor c was also found to predict group performance in diverse tasks in MBA classes lasting over several months. Thereby, highly collectively intelligent groups earned significantly higher scores on their group assignments although their members did not do any better on other individually performed assignments. Moreover, highly collective intelligent teams improved performance over time suggesting that more collectively intelligent teams learn better. This is another potential parallel to individual intelligence where more intelligent people are found to acquire new material quicker.
Individual intelligence can be used to predict plenty of life outcomes from school attainment and career success to health outcomes and even mortality. Whether collective intelligence is able to predict other outcomes besides group performance on mental tasks has still to be investigated.
Potential connections to individual intelligence
Gladwell (2008) showed that the relationship between individual IQ and success works only to a certain point and that additional IQ points over an estimate of IQ 120 do not translate into real life advantages. If a similar border exists for Group-IQ or if advantages are linear and infinite, has still to be explored. Similarly, demand for further research on possible connections of individual and collective intelligence exists within plenty of other potentially transferable logics of individual intelligence, such as, for instance, the development over time or the question of improving intelligence. Whereas it is controversial whether human intelligence can be enhanced via training, a group's collective intelligence potentially offers simpler opportunities for improvement by exchanging team members or implementing structures and technologies. Moreover, social sensitivity was found to be, at least temporarily, improvable by reading literary fiction as well as watching drama movies. In how far such training ultimately improves collective intelligence through social sensitivity remains an open question.
There are further more advanced concepts and factor models attempting to explain individual cognitive ability including the categorization of intelligence in fluid and crystallized intelligence or the hierarchical model of intelligence differences. Further supplementing explanations and conceptualizations for the factor structure of the Genomes of collective intelligence besides a general 'c factor', though, are missing yet.
Controversies and Counter-Evidence for Collective Intelligence
Other scholars explain team performance by aggregating team members' general intelligence to the team level instead of building an own overall collective intelligence measure. Devine and Philips (2001) showed in a meta-analysis that mean cognitive ability predicts team performance in laboratory settings (0.37) as well as field settings (0.14) – note that this is only a small effect. Suggesting a strong dependence on the relevant tasks, other scholars showed that tasks requiring a high degree of communication and cooperation are found to be most influenced by the team member with the lowest cognitive ability. Tasks in which selecting the best team member is the most successful strategy, are shown to be most influenced by the member with the highest cognitive ability.
Since Woolley et al.'s results do not show any influence of group satisfaction, group cohesiveness, or motivation, they, at least implicitly, challenge these concepts regarding the importance for group performance in general and thus contrast meta-analytically proven evidence concerning the positive effects of group cohesion, motivation and satisfaction on group performance.
Some scholars have noted that the evidence for collective intelligence in the body of work by Wolley et al. is weak and may contain errors or misunderstandings of the data. For example, Woolley et al. stated in their findings that the maximum individual score on the Wonderlic Personnel Test (WPT; an individual intelligence test used in their research) was 39, but also that the maximum averaged team score on the same test was also a 39. This indicates that their sample seemingly had a team composed entirely of people who, individually, got exactly the same score on the WPT, and also all happened to all have achieved the highest scores on the WPT found in Woolley et al. This was noted by scholars as particularly unlikely to occur. Other anomalies found in the data indicate that results may be driven in part by low-effort responding. For instance, Woolley et al.'s data indicates that at least one team scored a 0 on a task in which they were given 10 minutes to come up with as many uses for a brick as possible. Similarly, Woolley et al.'s data show that at least one team had an average score of 8 out of 50 on the WPT. Scholars have noted that the probability of this occurring with study participants who are putting forth effort is nearly zero. This may explain why Woolley et al. found that the group's individual intelligence scores were not predictive of performance. In addition, low effort on tasks in human subjects research may inflate evidence for a supposed collective intelligence factor based on similarity of performance across tasks, because a team's low effort on one research task may generalize to low effort across many tasks. It is notable that such a phenomenon is present merely because of the low stakes setting of laboratory research for research participants and not because it reflects how teams operate in organizations.
Noteworthy is also that the involved researchers among the confirming findings widely overlap with each other and with the authors participating in the original first study around Anita Woolley.
On 3 May 2022, the authors of "Quantifying collective intelligence in human groups", who include Riedl and Woolley from the original 2010 paper on Collective Intelligence, issued a correction to the article after mathematically impossible findings reported in the article were noted publicly by researcher Marcus Credé. Among the corrections is an admission that the average variance extracted (AVE)--that is to say, the evidence for collective intelligence—was only 19.6% from their Confirmatory Factor Analysis. Notable is that an AVE of at least 50% is generally required to demonstrate evidence for convergent validity of a single factor, with greater than 70% generally indicating good evidence for the factor. Therefore, the evidence for collective intelligence referred to as "robust" in Riedl et al. is in fact quite weak or nonexistent, as their primary evidence does not meet or near even the lowest thresholds of acceptable evidence for a latent factor. Curiously, despite this and several other factual inaccuracies found throughout the article, the paper has not been retracted, and these inaccuracies were apparently not originally detected by the author team, peer reviewers, or editors of the journal.
Alternative mathematical techniques
Computational collective intelligence

In 2001, Tadeusz (Tad) Szuba from the AGH University in Poland proposed a formal model for the phenomenon of collective intelligence. It is assumed to be an unconscious, random, parallel, and distributed computational process, run in mathematical logic by the social structure.
In this model, beings and information are modeled as abstract information molecules carrying expressions of mathematical logic. They are quasi-randomly displacing due to their interaction with their environments with their intended displacements. Their interaction in abstract computational space creates multi-thread inference process which we perceive as collective intelligence. Thus, a non-Turing model of computation is used. This theory allows simple formal definition of collective intelligence as the property of social structure and seems to be working well for a wide spectrum of beings, from bacterial colonies up to human social structures. Collective intelligence considered as a specific computational process is providing a straightforward explanation of several social phenomena. For this model of collective intelligence, the formal definition of IQS (IQ Social) was proposed and was defined as "the probability function over the time and domain of N-element inferences which are reflecting inference activity of the social structure". While IQS seems to be computationally hard, modeling of social structure in terms of a computational process as described above gives a chance for approximation. Prospective applications are optimization of companies through the maximization of their IQS, and the analysis of drug resistance against collective intelligence of bacterial colonies.
Collective intelligence quotient
One measure sometimes applied, especially by more artificial intelligence focused theorists, is a "collective intelligence quotient" (or "cooperation quotient") – which can be normalized from the "individual" intelligence quotient (IQ) – thus making it possible to determine the marginal intelligence added by each new individual participating in the collective action, thus using metrics to avoid the hazards of group think and stupidity.
Applications
There have been many recent applications of collective intelligence, including in fields such as crowd-sourcing, citizen science and prediction markets. The Nesta Centre for Collective Intelligence Design was launched in 2018 and has produced many surveys of applications as well as funding experiments. In 2020 the UNDP Accelerator Labs began using collective intelligence methods in their work to accelerate innovation for the Sustainable Development Goals.
Elicitation of point estimates
Here, the goal is to get an estimate (in a single value) of something. For example, estimating the weight of an object, or the release date of a product or probability of success of a project etc. as seen in prediction markets like Intrade, HSX or InklingMarkets and also in several implementations of crowdsourced estimation of a numeric outcome such as the Delphi method. Essentially, we try to get the average value of the estimates provided by the members in the crowd.
Opinion aggregation
In this situation, opinions are gathered from the crowd regarding an idea, issue or product. For example, trying to get a rating (on some scale) of a product sold online (such as Amazon's star rating system). Here, the emphasis is to collect and simply aggregate the ratings provided by customers/users.
Idea Collection
In these problems, someone solicits ideas for projects, designs or solutions from the crowd. For example, ideas on solving a data science problem (as in Kaggle) or getting a good design for a T-shirt (as in Threadless) or in getting answers to simple problems that only humans can do well (as in Amazon's Mechanical Turk). The objective is to gather the ideas and devise some selection criteria to choose the best ideas.
James Surowiecki divides the advantages of disorganized decision-making into three main categories, which are cognition, cooperation and coordination.
Cognition
Market judgment
Because of the Internet's ability to rapidly convey large amounts of information throughout the world, the use of collective intelligence to predict stock prices and stock price direction has become increasingly viable. Websites aggregate stock market information that is as current as possible so professional or amateur stock analysts can publish their viewpoints, enabling amateur investors to submit their financial opinions and create an aggregate opinion. The opinion of all investor can be weighed equally so that a pivotal premise of the effective application of collective intelligence can be applied: the masses, including a broad spectrum of stock market expertise, can be utilized to more accurately predict the behavior of financial markets.
Collective intelligence underpins the efficient-market hypothesis of Eugene Fama – although the term collective intelligence is not used explicitly in his paper. Fama cites research conducted by Michael Jensen in which 89 out of 115 selected funds underperformed relative to the index during the period from 1955 to 1964. But after removing the loading charge (up-front fee) only 72 underperformed while after removing brokerage costs only 58 underperformed. On the basis of such evidence index funds became popular investment vehicles using the collective intelligence of the market, rather than the judgement of professional fund managers, as an investment strategy.
Predictions in politics and technology

Political parties mobilize large numbers of people to form policy, select candidates and finance and run election campaigns. Knowledge focusing through various voting methods allows perspectives to converge through the assumption that uninformed voting is to some degree random and can be filtered from the decision process leaving only a residue of informed consensus. Critics point out that often bad ideas, misunderstandings, and misconceptions are widely held, and that structuring of the decision process must favor experts who are presumably less prone to random or misinformed voting in a given context.
Companies such as Affinnova (acquired by Nielsen), Google, InnoCentive, Marketocracy, and Threadless have successfully employed the concept of collective intelligence in bringing about the next generation of technological changes through their research and development (R&D), customer service, and knowledge management. An example of such application is Google's Project Aristotle in 2012, where the effect of collective intelligence on team makeup was examined in hundreds of the company's R&D teams.
Cooperation
Networks of trust

In 2012, the Global Futures Collective Intelligence System (GFIS) was created by The Millennium Project, which epitomizes collective intelligence as the synergistic intersection among data/information/knowledge, software/hardware, and expertise/insights that has a recursive learning process for better decision-making than the individual players alone.
New media are often associated with the promotion and enhancement of collective intelligence. The ability of new media to easily store and retrieve information, predominantly through databases and the Internet, allows for it to be shared without difficulty. Thus, through interaction with new media, knowledge easily passes between sources resulting in a form of collective intelligence. The use of interactive new media, particularly the internet, promotes online interaction and this distribution of knowledge between users.
Francis Heylighen, Valentin Turchin, and Gottfried Mayer-Kress are among those who view collective intelligence through the lens of computer science and cybernetics. In their view, the Internet enables collective intelligence at the widest, planetary scale, thus facilitating the emergence of a global brain.
The developer of the World Wide Web, Tim Berners-Lee, aimed to promote sharing and publishing of information globally. Later his employer opened up the technology for free use. In the early '90s, the Internet's potential was still untapped, until the mid-1990s when 'critical mass', as termed by the head of the Advanced Research Project Agency (ARPA), Dr. J.C.R. Licklider, demanded more accessibility and utility. The driving force of this Internet-based collective intelligence is the digitization of information and communication. Henry Jenkins, a key theorist of new media and media convergence draws on the theory that collective intelligence can be attributed to media convergence and participatory culture. He criticizes contemporary education for failing to incorporate online trends of collective problem solving into the classroom, stating "whereas a collective intelligence community encourages ownership of work as a group, schools grade individuals". Jenkins argues that interaction within a knowledge community builds vital skills for young people, and teamwork through collective intelligence communities contribute to the development of such skills. Collective intelligence is not merely a quantitative contribution of information from all cultures, it is also qualitative.
Lévy and de Kerckhove consider CI from a mass communications perspective, focusing on the ability of networked information and communication technologies to enhance the community knowledge pool. They suggest that these communications tools enable humans to interact and to share and collaborate with both ease and speed. With the development of the Internet and its widespread use, the opportunity to contribute to knowledge-building communities, such as Wikipedia, is greater than ever before. These computer networks give participating users the opportunity to store and to retrieve knowledge through the collective access to these databases and allow them to "harness the hive" Researchers at the MIT Center for Collective Intelligence research and explore collective intelligence of groups of people and computers.
In this context collective intelligence is often confused with shared knowledge. The former is the sum total of information held individually by members of a community while the latter is information that is believed to be true and known by all members of the community. Collective intelligence as represented by Web 2.0 has less user engagement than collaborative intelligence. An art project using Web 2.0 platforms is "Shared Galaxy", an experiment developed by an anonymous artist to create a collective identity that shows up as one person on several platforms like MySpace, Facebook, YouTube and Second Life. The password is written in the profiles and the accounts named "Shared Galaxy" are open to be used by anyone. In this way many take part in being one. Another art project using collective intelligence to produce artistic work is Curatron, where a large group of artists together decides on a smaller group that they think would make a good collaborative group. The process is used based on an algorithm computing the collective preferences In creating what he calls 'CI-Art', Nova Scotia based artist Mathew Aldred follows Pierry Lévy's definition of collective intelligence. Aldred's CI-Art event in March 2016 involved over four hundred people from the community of Oxford, Nova Scotia, and internationally. Later work developed by Aldred used the UNU swarm intelligence system to create digital drawings and paintings. The Oxford Riverside Gallery (Nova Scotia) held a public CI-Art event in May 2016, which connected with online participants internationally.

In social bookmarking (also called collaborative tagging), users assign tags to resources shared with other users, which gives rise to a type of information organisation that emerges from this crowdsourcing process. The resulting information structure can be seen as reflecting the collective knowledge (or collective intelligence) of a community of users and is commonly called a "Folksonomy", and the process can be captured by models of collaborative tagging.
Recent research using data from the social bookmarking website Delicious, has shown that collaborative tagging systems exhibit a form of complex systems (or self-organizing) dynamics. Although there is no central controlled vocabulary to constrain the actions of individual users, the distributions of tags that describe different resources has been shown to converge over time to a stable power law distributions. Once such stable distributions form, examining the correlations between different tags can be used to construct simple folksonomy graphs, which can be efficiently partitioned to obtained a form of community or shared vocabularies. Such vocabularies can be seen as a form of collective intelligence, emerging from the decentralised actions of a community of users. The Wall-it Project is also an example of social bookmarking.
P2P business
Research performed by Tapscott and Williams has provided a few examples of the benefits of collective intelligence to business:
- Talent utilization
- At the rate technology is changing, no firm can fully keep up in the innovations needed to compete. Instead, smart firms are drawing on the power of mass collaboration to involve participation of the people they could not employ. This also helps generate continual interest in the firm in the form of those drawn to new idea creation as well as investment opportunities.
- Demand creation
- Firms can create a new market for complementary goods by engaging in open-source community. Firms also are able to expand into new fields that they previously would not have been able to without the addition of resources and collaboration from the community. This creates, as mentioned before, a new market for complementary goods for the products in said new fields.
- Costs reduction
- Mass collaboration can help to reduce costs dramatically. Firms can release a specific software or product to be evaluated or debugged by online communities. The results will be more personal, robust and error-free products created in a short amount of time and costs. New ideas can also be generated and explored by collaboration of online communities creating opportunities for free R&D outside the confines of the company.
Open-source software
Cultural theorist and online community developer, John Banks considered the contribution of online fan communities in the creation of the Trainz product. He argued that its commercial success was fundamentally dependent upon "the formation and growth of an active and vibrant online fan community that would both actively promote the product and create content- extensions and additions to the game software".
The increase in user created content and interactivity gives rise to issues of control over the game itself and ownership of the player-created content. This gives rise to fundamental legal issues, highlighted by Lessig and Bray and Konsynski, such as intellectual property and property ownership rights.
Gosney extends this issue of Collective Intelligence in videogames one step further in his discussion of alternate reality gaming. This genre, he describes as an "across-media game that deliberately blurs the line between the in-game and out-of-game experiences" as events that happen outside the game reality "reach out" into the player's lives in order to bring them together. Solving the game requires "the collective and collaborative efforts of multiple players"; thus the issue of collective and collaborative team play is essential to ARG. Gosney argues that the Alternate Reality genre of gaming dictates an unprecedented level of collaboration and "collective intelligence" in order to solve the mystery of the game.
Benefits of co-operation
Co-operation helps to solve most important and most interesting multi-science problems. In his book, James Surowiecki mentioned that most scientists think that benefits of co-operation have much more value when compared to potential costs. Co-operation works also because at best it guarantees number of different viewpoints. Because of the possibilities of technology global co-operation is nowadays much easier and productive than before. It is clear that, when co-operation goes from university level to global it has significant benefits.
For example, why do scientists co-operate? Science has become more and more isolated and each science field has spread even more and it is impossible for one person to be aware of all developments. This is true especially in experimental research where highly advanced equipment requires special skills. With co-operation scientists can use information from different fields and use it effectively instead of gathering all the information just by reading by themselves."
Coordination
Ad-hoc communities
Military, trade unions, and corporations satisfy some definitions of CI – the most rigorous definition would require a capacity to respond to very arbitrary conditions without orders or guidance from "law" or "customers" to constrain actions. Online advertising companies are using collective intelligence to bypass traditional marketing and creative agencies.
The UNU open platform for "human swarming" (or "social swarming") establishes real-time closed-loop systems around groups of networked users molded after biological swarms, enabling human participants to behave as a unified collective intelligence. When connected to UNU, groups of distributed users collectively answer questions and make predictions in real-time. Early testing shows that human swarms can out-predict individuals. In 2016, an UNU swarm was challenged by a reporter to predict the winners of the Kentucky Derby, and successfully picked the first four horses, in order, beating 540 to 1 odds.
Specialized information sites such as Digital Photography Review or Camera Labs is an example of collective intelligence. Anyone who has an access to the internet can contribute to distributing their knowledge over the world through the specialized information sites.
In learner-generated context a group of users marshal resources to create an ecology that meets their needs often (but not only) in relation to the co-configuration, co-creation and co-design of a particular learning space that allows learners to create their own context. Learner-generated contexts represent an ad hoc community that facilitates coordination of collective action in a network of trust. An example of learner-generated context is found on the Internet when collaborative users pool knowledge in a "shared intelligence space". As the Internet has developed so has the concept of CI as a shared public forum. The global accessibility and availability of the Internet has allowed more people than ever to contribute and access ideas.
Games such as The Sims Series, and Second Life are designed to be non-linear and to depend on collective intelligence for expansion. This way of sharing is gradually evolving and influencing the mindset of the current and future generations. For them, collective intelligence has become a norm. In Terry Flew's discussion of 'interactivity' in the online games environment, the ongoing interactive dialogue between users and game developers, he refers to Pierre Lévy's concept of Collective Intelligence and argues this is active in videogames as clans or guilds in MMORPG constantly work to achieve goals. Henry Jenkins proposes that the participatory cultures emerging between games producers, media companies, and the end-users mark a fundamental shift in the nature of media production and consumption. Jenkins argues that this new participatory culture arises at the intersection of three broad new media trends. Firstly, the development of new media tools/technologies enabling the creation of content. Secondly, the rise of subcultures promoting such creations, and lastly, the growth of value adding media conglomerates, which foster image, idea and narrative flow.
Coordinating collective actions

Improvisational actors also experience a type of collective intelligence which they term "group mind", as theatrical improvisation relies on mutual cooperation and agreement, leading to the unity of "group mind".
Growth of the Internet and mobile telecom has also produced "swarming" or "rendezvous" events that enable meetings or even dates on demand. The full impact has yet to be felt but the anti-globalization movement, for example, relies heavily on e-mail, cell phones, pagers, SMS and other means of organizing. The Indymedia organization does this in a more journalistic way. Such resources could combine into a form of collective intelligence accountable only to the current participants yet with some strong moral or linguistic guidance from generations of contributors – or even take on a more obviously democratic form to advance shared goal.
A further application of collective intelligence is found in the "Community Engineering for Innovations". In such an integrated framework proposed by Ebner et al., idea competitions and virtual communities are combined to better realize the potential of the collective intelligence of the participants, particularly in open-source R&D. In management theory the use of collective intelligence and crowd sourcing leads to innovations and very robust answers to quantitative issues. Therefore, collective intelligence and crowd sourcing is not necessarily leading to the best solution to economic problems, but to a stable, good solution.
Coordination in different types of tasks
Collective actions or tasks require different amounts of coordination depending on the complexity of the task. Tasks vary from being highly independent simple tasks that require very little coordination to complex interdependent tasks that are built by many individuals and require a lot of coordination. In the article written by Kittur, Lee and Kraut the writers introduce a problem in cooperation: "When tasks require high coordination because the work is highly interdependent, having more contributors can increase process losses, reducing the effectiveness of the group below what individual members could optimally accomplish". Having a team too large the overall effectiveness may suffer even when the extra contributors increase the resources. In the end the overall costs from coordination might overwhelm other costs.
Group collective intelligence is a property that emerges through coordination from both bottom-up and top-down processes. In a bottom-up process the different characteristics of each member are involved in contributing and enhancing coordination. Top-down processes are more strict and fixed with norms, group structures and routines that in their own way enhance the group's collective work.
Alternative views
A tool for combating self-preservation
Tom Atlee reflects that, although humans have an innate ability to gather and analyze data, they are affected by culture, education and social institutions. A single person tends to make decisions motivated by self-preservation. Therefore, without collective intelligence, humans may drive themselves into extinction based on their selfish needs.
Separation from IQism
Phillip Brown and Hugh Lauder quotes Bowles and Gintis (1976) that in order to truly define collective intelligence, it is crucial to separate 'intelligence' from IQism. They go on to argue that intelligence is an achievement and can only be developed if allowed to. For example, earlier on, groups from the lower levels of society are severely restricted from aggregating and pooling their intelligence. This is because the elites fear that the collective intelligence would convince the people to rebel. If there is no such capacity and relations, there would be no infrastructure on which collective intelligence is built. This reflects how powerful collective intelligence can be if left to develop.
Artificial intelligence views
Skeptics, especially those critical of artificial intelligence and more inclined to believe that risk of bodily harm and bodily action are the basis of all unity between people, are more likely to emphasize the capacity of a group to take action and withstand harm as one fluid mass mobilization, shrugging off harms the way a body shrugs off the loss of a few cells. This train of thought is most obvious in the anti-globalization movement and characterized by the works of John Zerzan, Carol Moore, and Starhawk, who typically shun academics. These theorists are more likely to refer to ecological and collective wisdom and to the role of consensus process in making ontological distinctions than to any form of "intelligence" as such, which they often argue does not exist, or is mere "cleverness".
Harsh critics of artificial intelligence on ethical grounds are likely to promote collective wisdom-building methods, such as the new tribalists and the Gaians. Whether these can be said to be collective intelligence systems is an open question. Some, e.g. Bill Joy, simply wish to avoid any form of autonomous artificial intelligence and seem willing to work on rigorous collective intelligence in order to remove any possible niche for AI.
In contrast to these views, companies such as Amazon Mechanical Turk and CrowdFlower are using collective intelligence and crowdsourcing or consensus-based assessment to collect the enormous amounts of data for machine learning algorithms.