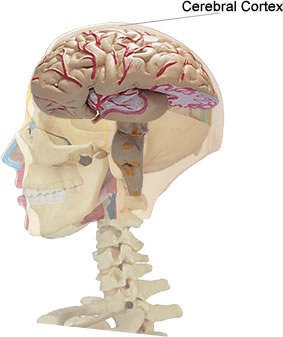
Analytical skill is the ability to deconstruct information into smaller categories in order to draw conclusions. Analytical skill consists of categories that include logical reasoning, critical thinking, communication, research, data analysis and creativity. Analytical skill is taught in contemporary education with the intention of fostering the appropriate practices for future professions. The professions that adopt analytical skill include educational institutions, public institutions, community organisations and industry.
Richards J. Heuer Jr. explained that
Thinking analytically is a skill like carpentry or driving a car. It can be taught, it can be learned, and it can improve with practice. But like many other skills, such as riding a bike, it is not learned by sitting in a classroom and being told how to do it. Analysts learn by doing.
In the article by Freed, the need for programs within the educational system to help students develop these skills is demonstrated. Workers "will need more than elementary basic skills to maintain the standard of living of their parents. They will have to think for a living, analyse problems and solutions, and work cooperatively in teams".
Logical Reasoning
Logical reasoning is a process consisting of inferences, where premises and hypotheses are formulated to arrive at a probable conclusion. It is a broad term covering three sub-classifications in deductive reasoning, inductive reasoning and abductive reasoning.
Deductive Reasoning
‘Deductive reasoning is a basic form of valid reasoning, commencing with a general statement or hypothesis, then examines the possibilities to reach a specific, logical conclusion’. This scientific method utilises deductions, to test hypotheses and theories, to predict if possible observations were correct.
A logical deductive reasoning sequence can be executed by establishing: an assumption, followed by another assumption and finally, conducting an inference. For example, ‘All men are mortal. Harold is a man. Therefore, Harold is mortal.’
For deductive reasoning to be upheld, the hypothesis must be correct, therefore, reinforcing the notion that the conclusion is logical and true. It is possible for deductive reasoning conclusions to be inaccurate or incorrect entirely, but the reasoning and premise is logical. For example, ‘All bald men are grandfathers. Harold is bald. Therefore, Harold is a grandfather.’ is a valid and logical conclusion but it is not true as the original assumption is incorrect. Deductive reasoning is an analytical skill used in many professions such as management, as the management team delegates tasks for day-to-day business operations.
Inductive Reasoning
Inductive reasoning compiles information and data to establish a general assumption that is suitable to the situation. Inductive reasoning commences with an assumption based on faithful data, leading to a generalised conclusion. For example, ‘All the swans I have seen are white. (Premise) Therefore all swans are white. (Conclusion)’. It is clear that the conclusion is incorrect, therefore, it is a weak argument. To strengthen the conclusion, it is made more probable, for example, ‘All the swans I have seen are white. (Premise) Therefore most swans are probably white (Conclusion)’. Inductive reasoning is an analytical skill common in many professions such as the corporate environment, where statistics and data are constantly analysed.

The 6 types of inductive reasoning
- Generalised: This manner utilises a premise on a sample set to extract a conclusion about a population.
- Statistical: This is a method that utilises statistics based on a large and viable random sample set that is quantifiable to strengthen conclusions and observations.
- Bayesian: This form adapts statistical reasoning to account for additional or new data.
- Analogical: This is a method that records on the foundations of shared properties between two groups, leading to a conclusion that they are also likely to share further properties.
- Predictive: This form of reasoning extrapolates a conclusion about the future based on a current or past sample.
- Causal inference: This method of reasoning is formed around a causal link between the premise and the conclusion.
Abductive reasoning
Abductive reasoning commences with layered hypotheses, which may be insufficient with evidence, leading to a conclusion that is most likely explanatory for the problem. It is a form of reasoning where the conductor chooses a hypothesis that would best suit the given data. For example, when a patient is ill, the doctor gathers a hypothesis from the patient's symptoms, or other evidence, that they deem factual and appropriate. The doctor will then go through a list of possible illnesses and will attempt to assign the appropriate illness. Abductive reasoning is characterised by its lack of completeness, in evidence, explanation or both. This form of reasoning can be creative, intuitive and revolutionary due to its instinctive design.
Critical Thinking
Critical thinking is a skill used to interpret and explain the data given. It is the ability to think cautiously and rationally to resolve problems. This thinking is achieved by supporting conclusions without biases, having reliable evidence and reasoning, and using appropriate data and information. Critical thinking is an imperative skill as it underpins contemporary living in areas such as education and professional careers, but it is not restricted to a specific area.
Critical thinking is used to solve problems, calculate the likelihood, make decisions, and formulate inferences. Critical thinking requires examining information, reflective thinking, using appropriate skills, and confidence in the quality of the information given to come to a conclusion or plan. Critical thinking includes being willing to change if better information becomes available. As a critical thinker individuals do not accept assumptions without further questioning the reliability of it with further research and analysing the results found.
Developing Critical Thinking
Critical thinking can be developed through establishing personal beliefs and values. It is critical that individuals are able to query authoritative bodies: teachers, specialists, textbooks, books, newspapers, television etc. Querying these authorities allow critical thinking ability to be developed as the individual gains their own freedom and wisdom to think about reality and contemporary society, revering from autonomy.
Developing Critical Thinking through Probability Models
Critical thinking can be developed through probability models, where individuals adhere to a logical, conceptual understanding of mathematics and emphasise investigation, problem-solving, mathematical literacy and the use of mathematical discourse. The student actively constructs their knowledge and understanding, while teaching models function as a mediator by actively testing the student through querying, challenging and assigning investigation tasks, ultimately, allowing the student to think in deeper ways about various concepts, ideas and mathematical contexts.
Communication
Communication is a process where individuals transfer information from one another. It is a complex system consisting of a listener interpreting the information, understanding it and then transferring it. Communication as an analytical skill includes communicating with confidence, clarity, and sticking with the point you are trying to communicate. It consists of verbal and non-verbal communication. Communication is an imperative component of analytical skill as it allows the individual to develop relationships, contribute to group decisions, organisational communication, and influence media and culture.

Verbal Communication
Verbal communication is interaction through words in linguistic form. Verbal communication consists of oral communication, written communication and sign language. It is an effective form of communication as the individuals sending and receiving the information are physically present, allowing immediate responses. In this form of communication, the sender uses words, spoken or written, to express the message to the individuals receiving the information.
Verbal communication is an essential analytical skill as it allows for the development of positive relationships among individuals. This positive relationship is attributed to the notion that verbal communication between individuals fosters a depth of understanding, empathy and versatility among them, providing each other with more attention. Verbal communication is a skill that is commonly used in professions such as the health sector, where healthcare workers are desired to possess strong interpersonal skills. Verbal communication has been linked to patient satisfaction. An effective strategy to improve verbal communication ability is through debating as is it fosters communication and critical thinking.
Non-verbal Communication
Non-verbal communication is commonly known as unspoken dialogue between individuals. It is a significant analytical skill as it allows individuals to distinguish true feelings, opinions and behaviours, as individuals are more likely to believe nonverbal cues as opposed to verbal expressions. Non-verbal communication is able to transcend communicational barriers such as race, ethnicity and sexual orientation.
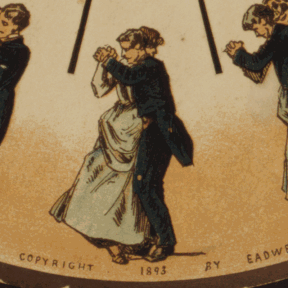
Statistical measures showcase that the true meaning behind all messages is 93% non-verbal and 7% verbal. Non-verbal communication is a critical analytical skill as it allows individuals to delve deeper into the meaning of messages. It allows individuals to analyse another person's perceptions, expressions and social beliefs. Individuals who excel in communicating and understanding non-verbal communication are able to analyse the interconnectedness of mutualism, social beliefs and expectations.
Communication Theories
A communication theory is an abstract understanding of how information is transferred from individuals. Many communication theories have been developed to foster and build upon the ongoing dynamic nature of how people communicate. Early models of communication were simple, such as Aristotle's model of communication, consisting of a speaker communicating a speech to an audience, leading to an effect. This is a basic form of communication that addresses communication as a linear concept where information is not being relayed back.
Modern theories for communication include Schramm's model where there are multiple individuals, each individual is encoding, interpreting and decoding the message, and messages are being transferred between one another. Schramm has included another factor in his model in experience i.e. expressing that each individual's experience influences their ability to interpret a message. Communication theories are constantly being developed to acclimatise to certain organisations or individuals. It is imperative for an individual to adopt a suitable communication theory for organisations to ensure that the organisation is able to function as desired. For example, traditional corporate hierarchy are commonly known to adopt a linear communicational model i.e. Aristotle's model of communication.
Research
Research is the construct of utilising tools and techniques to deconstruct and solve problems. While researching, it is important to distinguish what information is relevant to the data and avoiding excess, irrelevant data. Research involves the collection and analysis of information and data with the intention of founding new knowledge and/or deciphering a new understanding of existing data. Research ability is an analytical skill as it allows individuals to comprehend social implications. Research ability is valuable as it fosters transferable employment related skills. Research is primarily employed in academia and higher education, it is a profession pursued by many graduates, individuals intending to supervise or teach research students or those in pursuit of a PhD.
Research in Academia
In higher education, new research provides the most desired quality of evidence, if this is not available, then existing forms of evidence should be used. It is accepted that research provides the greatest form of knowledge, in the form of quantitative or qualitative data.
Research students are highly desired by various industries due to their dynamic mental capacity. Research students are commonly sought after due to their analysis and problem-solving ability, interpersonal and leadership skills, project management and organisation, research and information management and written and oral communication.
Data Analysis
Data analysis is a systematic method of cleaning, transforming and modelling statistical or logical techniques to describe and evaluate data. Using data analysis as an analytical skill means being able to examine large volumes of data and then identifying trends within the data. It is critical to be able to look at the data and determine what information is important and should be kept and what information is irrelevant and can be discarded. Data analysis includes finding different patterns within the information which allows you to narrow your research and come to a better conclusion. It is a tool to discover and decipher useful information for business decision-making. It is imperative in inferring information from data and adhering to a conclusion or decision from that data. Data analysis can stem from past or future data. Data analysis is an analytical skill, commonly adopted in business, as it allows organisations to become more efficient, internally and externally, solve complex problems and innovate.
Text Analysis
Text analysis is the discovery and understanding of valuable information in unstructured or large data. It is a method to transform raw data into business information, allowing for strategic business decisions by offering a method to extract and examine data, derive patterns and finally interpret the data.
Statistical Analysis
Statistical analysis involves the collection, analyses and presentation of data to decipher trends and patterns. It is common in research, industry and government to enhance the scientific aspects of the decision that needs to be made. It consists of descriptive analysis and inferential analysis.
Descriptive Analysis
Descriptive analysis provides information about a sample set that reflects the population by summarising relevant aspects of the dataset i.e. uncovering patterns. It displays the measures of central tendency and measures of spread, such as mean, deviation, proportion, frequency etc.
Inferential Analysis
Inferential analysis analyses a sample from complete data to compare the difference between treatment groups. Multiple conclusions are constructed by selecting different samples. Inferential analysis can provide evidence that, with a certain percentage of confidence, there is a relationship between two variables. It is adopted that the sample will be different to the population, thus, we further accept a degree of uncertainty.

Diagnostic Analysis
Diagnostic analysis showcases the origin of the problem by finding the cause from the insight found in statistical analysis. This form of analysis is useful to identify behavioural patterns of data.
Predictive Analysis
Predictive analysis is an advanced form of analytics that forecasts future activity, behaviour, trends and patterns from new and historical data. Its accuracy is based on how much faithful data is present and the degree of inference that can be exploited from it.
Prescriptive Analysis
Prescriptive analytics provide firms with optimal recommendations to solve complex decisions. It is used in many industries, such as aviation to optimise schedule selection for airline crew.
Creativity
