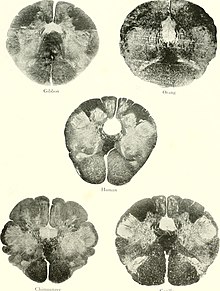
The evolution of the brain refers to the progressive development and complexity of neural structures over millions of years, resulting in the diverse range of brain sizes and functions observed across different species today, particularly in vertebrates.
The evolution of the brain has exhibited diverging adaptations within taxonomic classes, such as Mammalia, and even more diverse adaptations across other taxonomic classes. Brain-to-body size scales allometrically. This means that as body size changes, so do other physiological, anatomical, and biochemical connections between the brain and body. Small-bodied mammals tend to have relatively large brains compared to their bodies, while larger mammals (such as whales) have smaller brain-to-body ratios. When brain weight is plotted against body weight for primates, the regression line of the sample points can indicate the brain power of a species. For example, lemurs fall below this line, suggesting that for a primate of their size, a larger brain would be expected. In contrast, humans lie well above this line, indicating they are more encephalized than lemurs and, in fact, more encephalized than any other primate. This suggests that human brains have undergone a larger evolutionary increase in complexity relative to size. Some of these changes have been linked to multiple genetic factors, including proteins and other organelles.
Early history
One approach to understanding overall brain evolution is to use a paleoarchaeological timeline to trace the necessity for ever increasing complexity in structures that allow for chemical and electrical signaling. Because brains and other soft tissues do not fossilize as readily as mineralized tissues, scientists often look to other structures as evidence in the fossil record to get an understanding of brain evolution. This, however, leads to a dilemma as the emergence of organisms with more complex nervous systems with protective bone or other protective tissues that can then readily fossilize occur in the fossil record before evidence for chemical and electrical signaling. Evidence from 2008 showed that the ability to transmit electrical and chemical signals existed even before more complex multicellular lifeforms.
Fossilization of brain tissue, as well as other soft tissue, is nonetheless possible, and scientists can infer that the first brain structure appeared at least 521 million years ago, with fossil brain tissue present in sites of exceptional preservation.
Another approach to understanding brain evolution is to look at extant organisms that do not possess complex nervous systems, comparing anatomical features that allow for chemical or electrical messaging. For example, choanoflagellates are organisms that possess various membrane channels that are crucial to electrical signaling. The membrane channels of choanoflagellates' are homologous to the ones found in animal cells, and this is supported by the evolutionary connection between early choanoflagellates and the ancestors of animals. Another example of extant organisms with the capacity to transmit electrical signals would be the glass sponge, a multicellular organism, which is capable of propagating electrical impulses without the presence of a nervous system.
Before the evolutionary development of the brain, nerve nets, the simplest form of a nervous system developed. These nerve nets were a sort of precursor for the more evolutionarily advanced brains. They were first observed in Cnidaria and consist of a number of neurons spread apart that allow the organism to respond to physical contact. They are able to rudimentarily detect food and other chemicals, but these nerve nets do not allow them to detect the source of the stimulus.
Ctenophores also demonstrate this crude precursor to a brain or centralized nervous system, however they phylogenetically diverged before the phylum Porifera (the Sponges) and Cnidaria. There are two current theories on the emergence of nerve nets. One theory is that nerve nets may have developed independently in Ctenophores and Cnidarians. The other theory states that a common ancestor may have developed nerve nets, but they were lost in Porifera. While comparing the average neuron size and the packing density the difference between primate and mammal brains is shown.
A trend in brain evolution according to a study done with mice, chickens, monkeys and apes concluded that more evolved species tend to preserve the structures responsible for basic behaviors. A long term human study comparing the human brain to the primitive brain found that the modern human brain contains the primitive hindbrain region – what most neuroscientists call the protoreptilian brain. The purpose of this part of the brain is to sustain fundamental homeostatic functions, which are self regulating processes organisms use to help their bodies adapt. The pons and medulla are major structures found there. A new region of the brain developed in mammals about 250 million years after the appearance of the hindbrain. This region is known as the paleomammalian brain, the major parts of which are the hippocampi and amygdalas, often referred to as the limbic system. The limbic system deals with more complex functions including emotional, sexual and fighting behaviors. Of course, animals that are not vertebrates also have brains, and their brains have undergone separate evolutionary histories.
The brainstem and limbic system are largely based on nuclei, which are essentially balled-up clusters of tightly packed neurons and the axon fibers that connect them to each other, as well as to neurons in other locations. The other two major brain areas (the cerebrum and cerebellum) are based on a cortical architecture. At the outer periphery of the cortex, the neurons are arranged into layers (the number of which vary according to species and function) a few millimeters thick. There are axons that travel between the layers, but the majority of axon mass is below the neurons themselves. Since cortical neurons and most of their axon fiber tracts do not have to compete for space, cortical structures can scale more easily than nuclear ones. A key feature of cortex is that because it scales with surface area, more of it can be fit inside a skull by introducing convolutions, in much the same way that a dinner napkin can be stuffed into a glass by wadding it up. The degree of convolution is generally greater in species with more complex behavior, which benefits from the increased surface area.
The cerebellum, or "little brain," is behind the brainstem and below the occipital lobe of the cerebrum in humans. Its purposes include the coordination of fine sensorimotor tasks, and it may be involved in some cognitive functions, such as language and different motor skills that may involve hands and feet. The cerebellum helps keep equilibrium. Damage to the cerebellum would result in all physical roles in life to be affected. Human cerebellar cortex is finely convoluted, much more so than cerebral cortex. Its interior axon fiber tracts are called the arbor vitae, or Tree of Life.
The area of the brain with the greatest amount of recent evolutionary change is called the neocortex. In reptiles and fish, this area is called the pallium and is smaller and simpler relative to body mass than what is found in mammals. According to research, the cerebrum first developed about 200 million years ago. It is responsible for higher cognitive functions—for example, language, thinking, and related forms of information processing. It is also responsible for processing sensory input (together with the thalamus, a part of the limbic system that acts as an information router). The thalamus receives the different sensations before the information is then passed onto the cerebral cortex. Most of its function is subconscious, that is, not available for inspection or intervention by the conscious mind. The neocortex is an elaboration, or outgrowth, of structures in the limbic system, with which it is tightly integrated. The neocortex is the main part controlling many brain functions as it covers half of the whole brain in volume. The development of these recent evolutionary changes in the neocortex likely occurred as a result of new neural network formations and positive selections of certain genetic components.
Role of embryology
In addition to studying the fossil record, evolutionary history can be investigated via embryology. An embryo is an unborn/unhatched animal and evolutionary history can be studied by observing how processes in embryonic development are conserved (or not conserved) across species. Similarities between different species may indicate evolutionary connection. One way anthropologists study evolutionary connection between species is by observing orthologs. An ortholog is defined as two or more homologous genes between species that are evolutionarily related by linear descent. By using embryology the evolution of the brain can be tracked between various species.
Bone morphogenetic protein (BMP), a growth factor that plays a significant role in embryonic neural development, is highly conserved amongst vertebrates, as is sonic hedgehog (SHH), a morphogen that inhibits BMP to allow neural crest development. Tracking these growth factors with the use of embryology provides a deeper understanding of what areas of the brain diverged in their evolution. Varying levels of these growth factors lead to differing embryonic neural development which then in turn affects the complexity of future neural systems. Studying the brain's development at various embryonic stages across differing species provides additional insight into what evolutionary changes may have historically occurred. This then allows scientists to look into what factors may have caused such changes, such as links to neural network diversity, growth factor production, protein- coding selections, and other genetic factors.
Randomizing access and increasing size
Some animal phyla have gone through major brain enlargement through evolution (e.g. vertebrates and cephalopods both contain many lineages in which brains have grown through evolution) but most animal groups are composed only of species with extremely small brains. Some scientists argue that this difference is due to vertebrate and cephalopod neurons having evolved ways of communicating that overcome the scalability problem of neural networks while most animal groups have not. They argue that the reason why traditional neural networks fail to improve their function when they scale up is because filtering based on previously known probabilities cause self-fulfilling prophecy-like biases that create false statistical evidence giving a completely false worldview and that randomized access can overcome this problem and allow brains to be scaled up to more discriminating conditioned reflexes at larger brains that lead to new worldview forming abilities at certain thresholds. This means when neurons scale in a non randomized fashion that their functionality becomes more limited due to their neural networks being unable to process more complex systems without the exposure to new formations. This is explained by randomization allowing the entire brain to eventually get access to all information over the course of many shifts even though instant privileged access is physically impossible. They cite that vertebrate neurons transmit virus-like capsules containing RNA that are sometimes read in the neuron to which it is transmitted and sometimes passed further on unread which creates randomized access, and that cephalopod neurons make different proteins from the same gene which suggests another mechanism for randomization of concentrated information in neurons, both making it evolutionarily worth scaling up brains.
Brain re-organization
With the use of in vivo Magnetic resonance imaging (MRI) and tissue sampling, different cortical samples from members of each hominoid species were analyzed. In each species, specific areas were either relatively enlarged or shrunken, which can detail neural organizations. Different sizes in the cortical areas can show specific adaptations, functional specializations and evolutionary events that were changes in how the hominoid brain is organized. In early prediction it was thought that the frontal lobe, a large part of the brain that is generally devoted to behavior and social interaction, predicted the differences in behavior between hominoid and humans. Discrediting this theory was evidence supporting that damage to the frontal lobe in both humans and hominoids show atypical social and emotional behavior; thus, this similarity means that the frontal lobe was not very likely to be selected for reorganization. Instead, it is now believed that evolution occurred in other parts of the brain that are strictly associated with certain behaviors. The reorganization that took place is thought to have been more organizational than volumetric; whereas the brain volumes were relatively the same but specific landmark position of surface anatomical features, for example, the lunate sulcus suggest that the brains had been through a neurological reorganization. There is also evidence that the early hominin lineage also underwent a quiescent period, or a period of dormancy, which supports the idea of neural reorganization.
Dental fossil records for early humans and hominins show that immature hominins, including australopithecines and members of Homo, have a quiescent period (Bown et al. 1987). A quiescent period is a period in which there are no dental eruptions of adult teeth; at this time the child becomes more accustomed to social structure, and development of culture. During this time the child is given an extra advantage over other hominoids, devoting several years into developing speech and learning to cooperate within a community. This period is also discussed in relation to encephalization. It was discovered that chimpanzees do not have this neutral dental period, which suggests that a quiescent period occurred in very early hominin evolution. Using the models for neurological reorganization it can be suggested the cause for this period, dubbed middle childhood, is most likely for enhanced foraging abilities in varying seasonal environments.
Genetic factors in recent evolution
Genes involved in the neuro-development and in neuron physiology are extremely conserved between mammalian species (94% of genes expressed in common between humans and chimpanzees, 75% between humans and mice), compared to other organs. Therefore, few genes account for species differences in the human brain development and function.
Development of the human cerebral cortex
Main differences rely on the evolution of non-coding genomic regions, involved in the regulation of gene expression. This leads to differential expression of genes during the development of the human brain compared to other species, including chimpanzees. Some of these regions evolved fast in the human genome (human accelerated regions). The new genes expressed during human neurogenesis are notably associated with the NOTCH, WNT and mTOR pathways, but are also involved ZEB2, PDGFD and its receptor PDGFRβ. The human cerebral cortex is also characterized by a higher gradient of retinoic acid in the prefrontal cortex, leading to higher prefrontal cortex volume. All these differential gene expression lead to higher proliferation of the neural progenitors leading to more neurons in the human cerebral cortex. Some genes are lost in their expression during the development of the human cerebral cortex like GADD45G and FLRT2/FLRT3.
Another source of molecular novelty rely on new genes in the human or hominid genomes through segmental duplication. Around 30 new genes in the hominid genomes are dynamically expressed during human corticogenesis. Some were linked to higher proliferation of neural progenitors: NOTCH2NLA/B/C, ARHGAP11B, CROCCP2, TBC1D3, TMEM14B. Patients with deletions with NOTCH2NL genes display microcephaly, showing the necessity of such duplicated genes, acquired in the human genomes, in the proper corticogenesis.
MCPH1 and ASPM
Bruce Lahn, the senior author at the Howard Hughes Medical Center at the University of Chicago and colleagues have suggested that there are specific genes that control the size of the human brain. These genes continue to play a role in brain evolution, implying that the brain is continuing to evolve. The study began with the researchers assessing 214 genes that are involved in brain development. These genes were obtained from humans, macaques, rats and mice. Lahn and the other researchers noted points in the DNA sequences that caused protein alterations. These DNA changes were then scaled to the evolutionary time that it took for those changes to occur. The data showed the genes in the human brain evolved much faster than those of the other species. Once this genomic evidence was acquired, Lahn and his team decided to find the specific gene or genes that allowed for or even controlled this rapid evolution. Two genes were found to control the size of the human brain as it develops. These genes are Microcephalin (MCPH1) and Abnormal Spindle-like Microcephaly (ASPM). The researchers at the University of Chicago were able to determine that under the pressures of selection, both of these genes showed significant DNA sequence changes. Lahn's earlier studies displayed that Microcephalin experienced rapid evolution along the primate lineage which eventually led to the emergence of Homo sapiens. After the emergence of humans, Microcephalin seems to have shown a slower evolution rate. On the contrary, ASPM showed its most rapid evolution in the later years of human evolution once the divergence between chimpanzees and humans had already occurred.
Each of the gene sequences went through specific changes that led to the evolution of humans from ancestral relatives. In order to determine these alterations, Lahn and his colleagues used DNA sequences from multiple primates then compared and contrasted the sequences with those of humans. Following this step, the researchers statistically analyzed the key differences between the primate and human DNA to come to the conclusion, that the differences were due to natural selection. The changes in DNA sequences of these genes accumulated to bring about a competitive advantage and higher fitness that humans possess in relation to other primates. This comparative advantage is coupled with a larger brain size which ultimately allows the human mind to have a higher cognitive awareness.

ZEB2
ZEB2 is a protein- coding gene in the Homo sapien species. A 2021 study found that a delayed change in the shape of early brain cells causes the distinctly large human forebrain compared to other apes and identify ZEB2 as a genetic regulator of it, whose manipulation lead to acquisition of nonhuman ape cortical architecture in brain organoids.
NOVA1
In 2021, researchers reported that brain organoids created with stem cells into which they reintroduced the archaic gene variant NOVA1 present in Neanderthals and Denisovans via CRISPR-Cas9 shows that it has a major impact on neurodevelopment and that such genetic mutations during the evolution of the human brain underlie traits that separate modern humans from extinct Homo species. They found that expression of the archaic NOVA1 in cortical organoids leads to "modified synaptic protein interactions, affects glutamatergic signaling, underlies differences in neuronal connectivity, and promotes higher heterogeneity of neurons regarding their electrophysiological profiles". This research suggests positive selection of the modern NOVA1 gene, which may have promoted the randomization of neural scaling. A subsequent study failed to replicate the differences in organoid morphology between the modern human and the archaic NOVA1 variant, consistent with suspected unwanted side effects of CRISPR editing in the original study.
SRGAP2C and neuronal maturation
Less is known about neuronal maturation. Synaptic gene and protein expression are protracted, in line with the protracted synaptic maturation of human cortical neurons so called neoteny. This probably relies on the evolution of non-coding genomic regions. The consequence of the neoteny could be an extension of the period of synaptic plasticity and therefore of learning. A human-specific duplicated gene, SRGAP2C accounts for this synaptic neoteny and acts by regulating molecular pathways linked to neurodevelopmental disorders. Other genes are deferentially expressed in human neurons during their development such as osteocrin or cerebelin-2 .
LRRC37B and neuronal electrical properties
Even less is known about molecular specificities linked to the physiology of the human neurons. Human neurons are more divergent in the genes they express compared to chimpanzees than chimpanzees to gorilla, which suggests an acceleration of non-coding genomic regions associated with genes involved in neuronal physiology, in particular linked to the synapses. A hominid-specific duplicated gene, LRRC37B, codes for a transmembrane receptor that is selectively localized at the axon initial segment of human cortical pyramidal neurons. It inhibits their voltage-gated sodium channels that generate the action potentials leading to a lower neuronal excitability. Human cortical pyramidal neurons display a lower excitability compared to other mammalian species (including macaques and marmosets) which could lead to different circuit functions in the human species. Therefore, LRRC37B whose expression has been acquired in the human lineage after the separation from the chimpanzees could be a key gene in the function of the human cerebral cortex. LRRC37B binds to secreted FGF13A and SCN1B and modulate indirectly the activity of SCN8A, all involved in neural disorders such as epilepsy and autism. Therefore, LRRC37B may contribute to human-specific sensitivities to such disorders, both involved defects in neuronal excitability.
Genome repair
The genomic DNA of postmitotic neurons ordinarily does not replicate. Protection strategies have evolved to ensure the distinctive longevity of the neuronal genome. Human neurons are reliant on DNA repair processes to maintain function during an individual's life-time. DNA repair tends to occur preferentially at evolutionarily conserved sites that are specifically involved with the regulation of expression of genes essential for neuronal identity and function.
Other factors
Many other genetics may also be involved in recent evolution of the brain.
- For instance, scientists showed experimentally, with brain organoids grown from stem cells, how differences between humans and chimpanzees are also substantially caused by non-coding DNA (often discarded as relatively meaningless "junk DNA") – in particular via CRE-regulated expression of the ZNF558 gene for a transcription factor that regulates the SPATA18 gene. SPATA18 gene encodes a protein and is able to influence lysosome-like organelles that are found within mitochondria that eradicate oxidized mitochondrial proteins. This helps monitor the quality of the mitochondria as the disregulation of its quality control has been linked to cancer and degenerative diseases. This example may contribute to illustrations of the complexity and scope of relatively recent evolution to Homo sapiens.
- A change in gene TKTL1 could be a key factor of recent brain evolution and difference of modern humans to (other) apes and Neanderthals, related to neocortex-neurogenesis. However, the "archaic" allele attributed to Neanderthals is present in 0.03% of Homo sapiens, but no resultant phenotypic differences have been reported in these people. Additionally, as Herai et al. contend, more is not always better. In fact, enhanced neuron production "can lead to an abnormally enlarged cortex and layer-specific imbalances in glia/neuron ratios and neuronal subpopulations during neurodevelopment." Even the original study's authors agree that “any attempt to discuss prefrontal cortex and cognitive advantage of modern humans over Neandertals based on TKTL1 alone is problematic”.
- Some of the prior study's authors reported a similar ARHGAP11B mutation in 2016.
- Epigenetics also play a major role in the brain evolution in and to humans.
Recently evolved traits
- Language
A genome-wide association study meta-analysis reported genetic factors of, the so far uniquely human, language-related capacities, in particular factors of differences in skill-levels of five tested traits. It e.g. identified association with neuroanatomy of a language-related brain area via neuroimaging correlation. The data contributes to identifying or understanding the biological basis of this recently evolved characteristic capability.
Human brain evolution
One of the prominent ways of tracking the evolution of the human brain is through direct evidence in the form of fossils. The evolutionary history of the human brain shows primarily a gradually bigger brain relative to body size during the evolutionary path from early primates to hominids and finally to Homo sapiens. Because fossilized brain tissue is rare, a more reliable approach is to observe anatomical characteristics of the skull that offer insight into brain characteristics. One such method is to observe the endocranial cast (also referred to as endocasts). Endocasts occur when, during the fossilization process, the brain deteriorates away, leaving a space that is filled by surrounding sedimentary material over time. These casts, give an imprint of the lining of the brain cavity, which allows a visualization of what was there. This approach, however, is limited in regard to what information can be gathered. Information gleaned from endocasts is primarily limited to the size of the brain (cranial capacity or endocranial volume), prominent sulci and gyri, and size of dominant lobes or regions of the brain. While endocasts are extremely helpful in revealing superficial brain anatomy, they cannot reveal brain structure, particularly of deeper brain areas. By determining scaling metrics of cranial capacity as it relates to total number of neurons present in primates, it is also possible to estimate the number of neurons through fossil evidence.

Despite the limitations to endocasts, they can and do provide a basis for understanding human brain evolution, which shows primarily a gradually bigger brain. The evolutionary history of the human brain shows primarily a gradually bigger brain relative to body size during the evolutionary path from early primates to hominins and finally to Homo sapiens. This trend that has led to the present day human brain size indicates that there has been a 2-3 factor increase in size over the past 3 million years. This can be visualized with current data on hominin evolution, starting with Australopithecus, a group of hominins from which humans are likely descended. After all of the data, all observations concluded that the main development that occurred during evolution was the increase of brain size.
However, recent research has called into question the hypothesis of a threefold increase in brain size when comparing Homo sapiens with Australopithecus and chimpanzees. For example, in an article published in 2022 compiled a large data set of contemporary humans and found that the smallest human brains are less than twice that of large brained chimpanzees. As the authors write '...the upper limit of chimpanzee brain size is 500g/ml yet numerous modern humans have brain size below 900 g/ml.' (Note that in this quote, the unit g/ml is to be understood not in the usual way as gram per millilitre but rather as gram or millilitre. This is consistent because brain density is close to 1 g/ml.) Consequently, the authors argue that the notion of an increase in brain size being related to advances in cognition needs to be re-thought in light of global variation in brain size, as the brains of many modern humans with normal cognitive capacities are only 400g/ml larger than chimpanzees. Additionally, much of the increase in brain size - which occurs to a much greater degree in specific modern populations - can be explained by increases in correlated body size related to diet and climatic factors.
Australopiths lived from 3.85 to 2.95 million years ago with the general cranial capacity somewhere near that of the extant chimpanzee—around 300–500 cm3. Considering that the volume of the modern human brain is around 1,352 cm3 on average this represents a substantial amount of brain mass evolved. Australopiths are estimated to have a total neuron count of ~30-35 billion.
Progressing along the human ancestral timeline, brain size continues to steadily increase (see Homininae) when moving into the era of Homo. For example, Homo habilis, living 2.4 million to 1.4 million years ago and argued to be the first Homo species based on a host of characteristics, had a cranial capacity of around 600 cm3. Homo habilis is estimated to have had ~40 billion neurons.
A little closer to present day, Homo heidelbergensis lived from around 700,000 to 200,000 years ago and had a cranial capacity of around 1290 cm3 and having around 76 billion neurons.
Homo neaderthalensis, living 400,000 to 40,000 years ago, had a cranial capacity comparable to that of modern humans at around 1500–1600 cm3on average, with some specimens of Neanderthal having even greater cranial capacity. Neanderthals are estimated to have had around 85 billion neurons. The increase in brain size topped with Neanderthals, possibly due to their larger visual systems.
It is also important to note that the measure of brain mass or volume, seen as cranial capacity, or even relative brain size, which is brain mass that is expressed as a percentage of body mass, are not a measure of intelligence, use, or function of regions of the brain. Total neurons, however, also do not indicate a higher ranking in cognitive abilities. Elephants have a higher number of total neurons (257 billion) compared to humans (100 billion). Relative brain size, overall mass, and total number of neurons are only a few metrics that help scientists follow the evolutionary trend of increased brain to body ratio through the hominin phylogeny.
In 2021, scientists suggested that the brains of early Homo from Africa and Dmanisi, Georgia, Western Asia "retained a great ape-like structure of the frontal lobe" for far longer than previously thought – until about 1.5 million years ago. Their findings imply that Homo first dispersed out of Africa before human brains evolved to roughly their modern anatomical structure in terms of the location and organization of individual brain regions. It also suggests that this evolution occurred – not during – but only long after the Homo lineage evolved ~2.5 million years ago and after they – Homo erectus in particular – evolved to walk upright. What is the least controversial is that the brain expansion started about 2.6 Ma (about the same as the start of the Pleistocene), and ended around 0.2 Ma.
Evolution of the neocortex
In addition to just the size of the brain, scientists have observed changes in the folding of the brain, as well as in the thickness of the cortex. The more convoluted the surface of the brain is, the greater the surface area of the cortex which allows for an expansion of cortex. It is the most evolutionarily advanced part of the brain. Greater surface area of the brain is linked to higher intelligence as is the thicker cortex but there is an inverse relationship—the thicker the cortex, the more difficult it is for it to fold. In adult humans, thicker cerebral cortex has been linked to higher intelligence.
The neocortex is the most advanced and most evolutionarily young part of the human brain. It is six layers thick and is only present in mammals. It is especially prominent in humans and is the location of most higher level functioning and cognitive ability. The six-layered neocortex found in mammals is evolutionarily derived from a three-layer cortex present in all modern reptiles. This three-layer cortex is still conserved in some parts of the human brain such as the hippocampus and is believed to have evolved in mammals to the neocortex during the transition between the Triassic and Jurassic periods. After looking at history, the mammals had little neocortex compared to the primates as they had more cortex. The three layers of this reptilian cortex correlate strongly to the first, fifth and sixth layers of the mammalian neocortex. Across species of mammals, primates have greater neuronal density compared to rodents of similar brain mass and this may account for increased intelligence.
Theories of human brain evolution
Explanations of the rapid evolution and exceptional size of the human brain can be classified into five groups: instrumental, social, environmental, dietary, and anatomo-physiological. The instrumental hypotheses are based on the logic that evolutionary selection for larger brains is beneficial for species survival, dominance, and spread, because larger brains facilitate food-finding and mating success. The social hypotheses suggest that social behavior stimulates evolutionary expansion of brain size. Similarly, the environmental hypotheses suppose that encephalization is promoted by environmental factors such as stress, variability, and consistency. The dietary theories maintain that food quality and certain nutritional components directly contributed to the brain growth in the Homo genus. The anatomo-physiologic concepts, such as cranio-cerebral vascular hypertension due to head-down posture of the anthropoid fetus during pregnancy, are primarily focused on anatomic-functional changes that predispose to brain enlargement.
No single theory can completely account for human brain evolution. Multiple selective pressures in combination seems to have been involved. Synthetic theories have been proposed, but have not clearly explained reasons for the uniqueness of the human brain. Puzzlingly, brain enlargement has been found to have occurred independently in different primate lineages, but only human lineage ended up with an exceptional brain capacity. Fetal head-down posture may be an explanation of this conundrum because Homo sapiens is the only primate obligatory biped with upright posture.