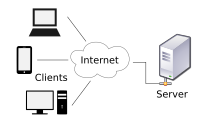


A server is a computer that provides information to other computers called "clients" on a computer network. This architecture is called the client–server model. Servers can provide various functionalities, often called "services", such as sharing data or resources among multiple clients or performing computations for a client. A single server can serve multiple clients, and a single client can use multiple servers. A client process may run on the same device or may connect over a network to a server on a different device. Typical servers are database servers, file servers, mail servers, print servers, web servers, game servers, and application servers.
Client–server systems are usually most frequently implemented by (and often identified with) the request–response model: a client sends a request to the server, which performs some action and sends a response back to the client, typically with a result or acknowledgment. Designating a computer as "server-class hardware" implies that it is specialized for running servers on it. This often implies that it is more powerful and reliable than standard personal computers, but alternatively, large computing clusters may be composed of many relatively simple, replaceable server components.
History
The use of the word server in computing comes from queueing theory, where it dates to the mid 20th century, being notably used in Kendall (1953) (along with "service"), the paper that introduced Kendall's notation. In earlier papers, such as the Erlang (1909), more concrete terms such as "[telephone] operators" are used.
In computing, "server" dates at least to RFC 5 (1969), one of the earliest documents describing ARPANET (the predecessor of Internet), and is contrasted with "user", distinguishing two types of host: "server-host" and "user-host". The use of "serving" also dates to early documents, such as RFC 4, contrasting "serving-host" with "using-host".
The Jargon File defines server in the common sense of a process performing service for requests, usually remote, with the 1981 version reading:
SERVER n. A kind of DAEMON which performs a service for the requester, which often runs on a computer other than the one on which the server runs.
The average utilization of a server in the early 2000s was 5 to 15%, but with the adoption of virtualization this figure started to increase to reduce the number of servers needed.
Operation

Strictly speaking, the term server refers to a computer program or process (running program). Through metonymy, it refers to a device used for (or a device dedicated to) running one or several server programs. On a network, such a device is called a host. In addition to server, the words serve and service (as verb and as noun respectively) are frequently used, though servicer and servant are not. The word service (noun) may refer to the abstract form of functionality, e.g. Web service. Alternatively, it may refer to a computer program that turns a computer into a server, e.g. Windows service. Originally used as "servers serve users" (and "users use servers"), in the sense of "obey", today one often says that "servers serve data", in the same sense as "give". For instance, web servers "serve [up] web pages to users" or "service their requests".
The server is part of the client–server model; in this model, a server serves data for clients. The nature of communication between a client and server is request and response. This is in contrast with peer-to-peer model in which the relationship is on-demand reciprocation. In principle, any computerized process that can be used or called by another process (particularly remotely, particularly to share a resource) is a server, and the calling process or processes is a client. Thus any general-purpose computer connected to a network can host servers. For example, if files on a device are shared by some process, that process is a file server. Similarly, web server software can run on any capable computer, and so a laptop or a personal computer can host a web server.
While request–response is the most common client-server design, there are others, such as the publish–subscribe pattern. In the publish-subscribe pattern, clients register with a pub-sub server, subscribing to specified types of messages; this initial registration may be done by request-response. Thereafter, the pub-sub server forwards matching messages to the clients without any further requests: the server pushes messages to the client, rather than the client pulling messages from the server as in request-response.
Purpose
The role of a server is to share data as well as to share resources and distribute work. A server computer can serve its own computer programs as well; depending on the scenario, this could be part of a quid pro quo transaction, or simply a technical possibility. The following table shows several scenarios in which a server is used.
Server type | Purpose | Clients |
---|---|---|
Application server | Hosts application back ends that user clients (front ends, web apps or locally installed applications) in the network connect to and use. These servers do not need to be part of the World Wide Web; any local network would do. | Clients with a browser or a local front end, or a web server |
Catalog server | Maintains an index or table of contents of information that can be found across a large distributed network, such as computers, users, files shared on file servers, and web apps. Directory servers and name servers are examples of catalog servers. | Any computer program that needs to find something on the network, such a Domain member attempting to log in, an email client looking for an email address, or a user looking for a file |
Communications server | Maintains an environment needed for one communication endpoint (user or devices) to find other endpoints and communicate with them. It may or may not include a directory of communication endpoints and a presence detection service, depending on the openness and security parameters of the network | Communication endpoints (users or devices) |
Computing server | Shares vast amounts of computing resources, especially CPU and random-access memory, over a network. | Any computer program that needs more CPU power and RAM than a personal computer can probably afford. The client must be a networked computer; otherwise, there would be no client-server model. |
Database server | Maintains and shares any form of database (organized collections of data with predefined properties that may be displayed in a table) over a network. | Spreadsheets, accounting software, asset management software or virtually any computer program that consumes well-organized data, especially in large volumes |
Fax server | Shares one or more fax machines over a network, thus eliminating the hassle of physical access | Any fax sender or recipient |
File server | Shares files and folders, storage space to hold files and folders, or both, over a network | Networked computers are the intended clients, even though local programs can be clients |
Game server | Enables several computers or gaming devices to play multiplayer video games | Personal computers or gaming consoles |
Mail server | Makes email communication possible in the same way that a post office makes snail mail communication possible | Senders and recipients of email |
Media server | Shares digital video or digital audio over a network through media streaming (transmitting content in a way that portions received can be watched or listened to as they arrive, as opposed to downloading an entire file and then using it) | User-attended personal computers equipped with a monitor and a speaker |
Print server | Shares one or more printers over a network, thus eliminating the hassle of physical access | Computers in need of printing something |
Sound server | Enables computer programs to play and record sound, individually or cooperatively | Computer programs of the same computer and network clients. |
Proxy server | Acts as an intermediary between a client and a server, accepting incoming traffic from the client and sending it to the server. Reasons for doing so include content control and filtering, improving traffic performance, preventing unauthorized network access or simply routing the traffic over a large and complex network. | Any networked computer |
Virtual server | Shares hardware and software resources with other virtual servers. It exists only as defined within specialized software called hypervisor. The hypervisor presents virtual hardware to the server as if it were real physical hardware. Server virtualization allows for a more efficient infrastructure. | Any networked computer |
Web server | Hosts web pages. A web server is what makes the World Wide Web possible. Each website has one or more web servers. Also, each server can host multiple websites. | Computers with a web browser |
Almost the entire structure of the Internet is based upon a client–server model. High-level root nameservers, DNS, and routers direct the traffic on the internet. There are millions of servers connected to the Internet, running continuously throughout the world and virtually every action taken by an ordinary Internet user requires one or more interactions with one or more servers. There are exceptions that do not use dedicated servers; for example, peer-to-peer file sharing and some implementations of telephony (e.g. pre-Microsoft Skype).
Hardware

Hardware requirement for servers vary widely, depending on the server's purpose and its software. Servers often are more powerful and expensive than the clients that connect to them.
The name server is used both for the hardware and software pieces. For the hardware servers, it is usually limited to mean the high-end machines although software servers can run on a variety of hardwares.
Since servers are usually accessed over a network, many run unattended without a computer monitor or input device, audio hardware and USB interfaces. Many servers do not have a graphical user interface (GUI). They are configured and managed remotely. Remote management can be conducted via various methods including Microsoft Management Console (MMC), PowerShell, SSH and browser-based out-of-band management systems such as Dell's iDRAC or HP's iLo.
Large servers
Large traditional single servers would need to be run for long periods without interruption. Availability would have to be very high, making hardware reliability and durability extremely important. Mission-critical enterprise servers would be very fault tolerant and use specialized hardware with low failure rates in order to maximize uptime. Uninterruptible power supplies might be incorporated to guard against power failure. Servers typically include hardware redundancy such as dual power supplies, RAID disk systems, and ECC memory, along with extensive pre-boot memory testing and verification. Critical components might be hot swappable, allowing technicians to replace them on the running server without shutting it down, and to guard against overheating, servers might have more powerful fans or use water cooling. They will often be able to be configured, powered up and down, or rebooted remotely, using out-of-band management, typically based on IPMI. Server casings are usually flat and wide, and designed to be rack-mounted, either on 19-inch racks or on Open Racks.
These types of servers are often housed in dedicated data centers. These will normally have very stable power and Internet and increased security. Noise is also less of a concern, but power consumption and heat output can be a serious issue. Server rooms are equipped with air conditioning devices.
-
A server rack seen from the rear
-
Wikimedia Foundation servers as seen from the front
-
Wikimedia Foundation servers as seen from the rear
-
Wikimedia Foundation servers as seen from the rear
Clusters
A server farm or server cluster is a collection of computer servers maintained by an organization to supply server functionality far beyond the capability of a single device. Modern data centers are now often built of very large clusters of much simpler servers, and there is a collaborative effort, Open Compute Project around this concept.
Appliances
A class of small specialist servers called network appliances are generally at the low end of the scale, often being smaller than common desktop computers.
Mobile
A mobile server has a portable form factor, e.g. a laptop. In contrast to large data centers or rack servers, the mobile server is designed for on-the-road or ad hoc deployment into emergency, disaster or temporary environments where traditional servers are not feasible due to their power requirements, size, and deployment time. The main beneficiaries of so-called "server on the go" technology include network managers, software or database developers, training centers, military personnel, law enforcement, forensics, emergency relief groups, and service organizations. To facilitate portability, features such as the keyboard, display, battery (uninterruptible power supply, to provide power redundancy in case of failure), and mouse are all integrated into the chassis.
Operating systems

On the Internet, the dominant operating systems among servers are UNIX-like open-source distributions, such as those based on Linux and FreeBSD, with Windows Server also having a significant share. Proprietary operating systems such as z/OS and macOS Server are also deployed, but in much smaller numbers. Servers that run Linux are commonly used as Webservers or Databanks. Windows Servers are used for Networks that are made out of Windows Clients.
Specialist server-oriented operating systems have traditionally had features such as:
- GUI not available or optional
- Ability to reconfigure and update both hardware and software to some extent without restart
- Advanced backup facilities to permit regular and frequent online backups of critical data,
- Transparent data transfer between different volumes or devices
- Flexible and advanced networking capabilities
- Automation capabilities such as daemons in UNIX and services in Windows
- Tight system security, with advanced user, resource, data, and memory protection.
- Advanced detection and alerting on conditions such as overheating, processor and disk failure.
In practice, today many desktop and server operating systems share similar code bases, differing mostly in configuration.
Energy consumption
In 2010, data centers (servers, cooling, and other electrical infrastructure) were responsible for 1.1–1.5% of electrical energy consumption worldwide and 1.7–2.2% in the United States. One estimate is that total energy consumption for information and communications technology saves more than 5 times its carbon footprint in the rest of the economy by increasing efficiency.
Global energy consumption is increasing due to the increasing demand of data and bandwidth. Natural Resources Defense Council (NRDC) states that data centers used 91 billion kilowatt hours (kWh) electrical energy in 2013 which accounts to 3% of global electricity usage.
Environmental groups have placed focus on the carbon emissions of data centers as it accounts to 200 million metric tons of carbon dioxide in a year.