Alcoholic cardiomyopathy | |
---|---|
![]() | |
Specialty | Cardiology |
Alcoholic cardiomyopathy (ACM) is a disease in which the long-term consumption of alcohol leads to heart failure. ACM is a type of dilated cardiomyopathy. The heart is unable to pump blood efficiently, leading to heart failure. It can affect other parts of the body if the heart failure is severe. It is most common in males between the ages of 35 and 50.
Etiology
The causal relationship between alcohol consumption and cardiomyopathy and heart failure is unclear. Per the American Heart Association (AHA), alcohol is one of the leading causes of dilated cardiomyopathy. However, multiple longitudinal studies have shown a paradoxical lowering of dilated cardiomyopathy with modest-to-moderate alcohol consumption.
ACM is a type of heart disease that occurs due to chronic alcohol consumption. The etiology of ACM is multifactorial, with a combination of genetic, environmental, and lifestyle factors playing a role. The direct toxic effects of alcohol on the heart muscle cells (cardiomyocytes) are considered the primary cause of ACM. Chronic alcohol consumption leads to the accumulation of toxic metabolites, such as acetaldehyde and reactive oxygen species, in the heart muscle cells. These toxic substances can cause oxidative stress, inflammation, and damage to the cardiomyocytes, leading to the development of ACM.
Additionally, chronic alcohol consumption can lead to deficiencies in essential vitamins and minerals, such as thiamine, magnesium, and selenium, which are important for the proper functioning of the heart. Thiamine deficiency, in particular, is common in people with alcohol use disorder and can lead to a condition known as beriberi, which can damage the heart muscle. Furthermore, chronic alcohol consumption can also lead to other cardiovascular risk factors, such as high blood pressure, high cholesterol levels, and obesity, which can contribute to the development of ACM. Overall, the etiology of ACM is complex and involves various factors that can damage the heart muscle over time.
Signs and symptoms
Signs and symptoms of alcoholic cardiomyopathy are indistinguishable from those seen in other forms of cardiomyopathy. These symptoms can include the following:
- Ankle, feet, and leg swelling (edema)
- This occurs because of a phenomenon known as third spacing. Third spacing occurs because the heart is unable to pump the blood throughout the body, and thus the fluid pools up in your veins. The fluid then eventually leaves your veins and enters the interstitial space, causing swelling. Doctors will sometimes test for pitting edema by pressing their fingers against the swelling to see if any "pitting" occurs.
- Overall swelling
- Loss of appetite
- Shortness of breath (dyspnea), especially with activity
- Breathing difficulty while lying down
- This medical term for this symptoms is orthopnea, it occurs because fluid builds up in the posterior portion of both lungs, making it difficult to breathe.
- Fatigue, weakness, faintness
- Decreased alertness or concentration
- Cough containing mucus, or pink, frothy material
- Decreased urine output (oliguria)
- Need to urinate at night (nocturia)
- Heart palpitations (irregular heart beat)
- Rapid pulse (tachycardia)
The signs and symptoms of alcoholic cardiomyopathy (ACM) can vary depending on the severity of the condition. In the early stages, people with ACM may not experience any symptoms. However, as the condition progresses, they may experience symptoms such as fatigue, shortness of breath, palpitations, and swelling of the legs and ankles. They may also experience chest pain, dizziness, and fainting. In some cases, ACM can cause arrhythmias or irregular heartbeats, which can be life-threatening. In advanced cases, people with ACM may develop severe heart failure, which can cause symptoms such as severe shortness of breath, wheezing, and coughing. If left untreated, ACM can lead to life-threatening complications such as heart failure, arrhythmias, and sudden cardiac death. Therefore, it is important to seek medical attention if any of these symptoms are experienced, especially if there is a history of chronic alcohol consumption.
Pathophysiology
Alcohol-induced cardiac toxicity (AiCT) is characterized as either acute or chronic. It is believed that consumption of large amounts of alcohol leads to cardiac inflammation, which can be detected by finding large amounts of troponin in the serum. Chronic consumption of alcohol (defined as greater than 80 g per day for at least 5 years) can lead to multi-organ failure, including myocardial dysfunction. The exact pathophysiologic mechanism by which chronic consumption of alcohol causes DCM is not well understood, however it's believed that genetic mutation, and mitochondrial damage due to oxidative stress injury may play a role.
Diagnosis
Abnormal heart sounds, murmurs, ECG abnormalities, and enlarged heart on chest x-ray may lead to the diagnosis. Echocardiogram abnormalities and cardiac catheterization or angiogram to rule out coronary artery blockages, along with a history of alcohol abuse can confirm the diagnosis. It's important to note that part of diagnosing Chronic ACM is noting the absence of coronary artery disease. It's also worth noting that the diagnosis of ACM is largely a diagnosis of exclusion.
The diagnosis of alcoholic cardiomyopathy is typically made based on a combination of the patient's medical history, physical examination, and diagnostic tests. Firstly, the doctor will ask the patient about their alcohol consumption habits, as well as any symptoms they may have experienced, such as shortness of breath or swelling in the legs. They may also perform a physical examination to check for signs of heart failure, such as an enlarged heart or fluid buildup in the lungs.
In addition to the patient's medical history and physical exam, the diagnosis of alcoholic cardiomyopathy is often confirmed with various diagnostic tests. One of the most common tests is an echocardiogram, which uses ultrasound waves to create images of the heart and can detect abnormalities in the heart's structure and function. Other tests may include an electrocardiogram (ECG) to measure the heart's electrical activity, and blood tests to check for elevated levels of certain enzymes that may indicate heart damage. If the diagnosis is confirmed, treatment typically involves stopping alcohol consumption and managing heart failure symptoms through medications, lifestyle changes, and in severe cases, heart transplantation.
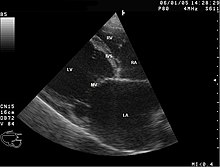
Prognosis
The prognosis is influenced by several factors, including the amount of alcohol and the time period over which it has been consumed, the presence or absence of dysrhythmias such as atrial fibrillation, and the width of the QRS complex. Some indications of poor prognosis include the following: patients with QRS > 120, patients who continue to consume alcohol for prolonged periods. Consumption of alcohol is directly related to the amount of alcohol consumed and length of consumption. Indicators of good prognosis include the following: successfully quitting the consumption of alcohol (associated with decreased hospital admissions), and patient compliance with beta blockers. Mortality is between 40–80% 10 years post-diagnosis.
The prognosis of alcoholic cardiomyopathy (ACM) varies depending on the severity of the condition, the extent of heart muscle damage, and the response to treatment. Without treatment, ACM can progress to severe heart failure, arrhythmias, and sudden cardiac death. However, with proper treatment, including cessation of alcohol consumption and management of heart failure symptoms, the prognosis can improve significantly.
Research has shown that the mortality rate for people with ACM is higher than that of the general population, with a five-year survival rate of around 50%. However, studies have also shown that people who stop drinking alcohol have a significantly better prognosis than those who continue to drink. In addition, people who receive early treatment for ACM, including medication and lifestyle modifications, have a better chance of improving their heart function and overall health.
The prognosis of ACM can also depend on the presence of other comorbidities such as diabetes, hypertension, and obesity. These conditions can exacerbate the effects of ACM on the heart and increase the risk of complications. Therefore, it is important to manage these comorbidities to improve the overall prognosis of ACM.
Complications
- Heart failure
- Cachexia
- Arrhythmias
- Cardioembolism
- Death
There are several complications that can arise as a result of alcoholic cardiomyopathy. For instance, individuals with this condition may be at a higher risk of developing blood clots, which can lead to heart attacks, strokes, or other serious cardiovascular events. Additionally, the weakened heart muscle may not be able to effectively pump blood to the lungs, leading to the accumulation of fluid in the lungs, a condition known as pulmonary edema.
Another potential complication of alcoholic cardiomyopathy is the development of arrhythmias, or abnormal heart rhythms. These irregular heart rhythms can range from mild to severe and may cause symptoms such as palpitations, lightheadedness, or even loss of consciousness. In some cases, arrhythmias can lead to sudden cardiac arrest, a life-threatening condition in which the heart suddenly stops.
Treatment
Treatment for alcoholic cardiomyopathy involves lifestyle changes, including complete abstinence from alcohol use, a low sodium diet, and fluid restriction, as well as medications. Medications may include ACE inhibitors, beta blockers, and diuretics which are commonly used in other forms of cardiomyopathy to reduce the strain on the heart. Persons with congestive heart failure may be considered for surgical insertion of an ICD or a pacemaker which can improve heart function. In cases where the heart failure is irreversible and worsening, heart transplant may be considered. Treatment will possibly prevent the heart from further deterioration, and the cardiomyopathy is largely reversible if complete abstinence from alcohol is maintained.
Unfortunately, for patients that require heart transplants, cardiomyopathy due to alcoholism has the lowest post-heart transplant survival out of all causes of cardiomyopathy. Per one study that compared 224 alcoholic cardiomyopathy patients to over 60,000 non-alcoholic cardiomyopathy patients, survival post heart transplant was less at 1 year, 5 years, 10 years, and 12 years.
Interestingly, in patients that are defined as "heavy drinkers" (defined as consuming >30g of alcohol/day) decreased alcohol consumption to moderate levels has been shown to be an effective treatment; in fact A retrospective cohort study analyzed data collected from over 3.8 million patients, and categorized patients as either abstinent drinkers, mild drinkers, moderate drinkers, and heavy drinkers. Despite having such a large sample size, the association between alcohol intake and cardiomyopathy remains unclear. The study found that patients that were either mild or moderate drinkers were the least likely to develop HF as compared to patients that were abstinent. The study also found that patients that increased their alcohol consumption from light to moderate and/or from moderate to heavy were at increased risk for heart failure. Although one might think that patients that were completely abstinent from alcohol would have would be least likely of being diagnosed with heart failure, it's actually patients categorized as either light or moderate drinkers had the lowest risk for developing HF.