Inquisitiveness and imagination will be hard to create any other way.
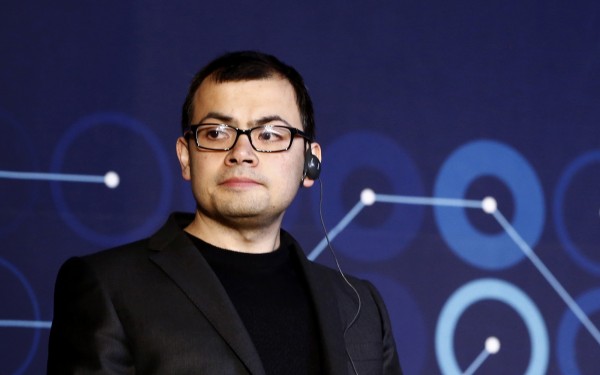
Currently, most AI systems are based on layers of mathematics that are only loosely inspired by the way the human brain works. But different types of machine learning, such as speech recognition or identifying objects in an image, require different mathematical structures, and the resulting algorithms are only able to perform very specific tasks.
Building AI that can perform general tasks, rather than niche ones, is a long-held desire in the world of machine learning. But the truth is that expanding those specialized algorithms to something more versatile remains an incredibly difficult problem, in part because human traits like inquisitiveness, imagination, and memory don’t exist or are only in their infancy in the world of AI.
In a paper published today in the journal Neuron, Hassabis and three coauthors argue that only by better understanding human intelligence can we hope to push the boundaries of what artificial intellects can achieve.
First, they say, better understanding of how the brain works will allow us to create new structures and algorithms for electronic intelligence. Second, lessons learned from building and testing cutting-edge AIs could help us better define what intelligence really is.
The paper itself reviews the history of neuroscience and artificial intelligence to understand the interactions between the two. It argues that deep learning, which uses layers of artificial neurons to understand inputs, and reinforcement learning, where systems learn by trial and error, both owe a great deal to neuroscience.
But it also points out that more recent advances haven’t leaned on biology as effectively, and that a general intelligence will need more human-like characteristics—such as an intuitive understanding of the real world and more efficient ways of learning. The solution, Hassabis and his colleagues argue, is a renewed “exchange of ideas between AI and neuroscience [that] can create a 'virtuous circle' advancing the objectives of both fields.”
Hassabis is not alone in this kind of thinking. Gary Marcus, a professor of psychology at New York University and former director of Uber’s AI lab, has argued that machine-learning systems could be improved using ideas gathered by studying the cognitive development of children.
Even so, implementing those findings digitally won’t be easy. As Hassabis explains in an interview with the Verge, artificial intelligence and neuroscience have become “two very, very large fields that are steeped in their own traditions,” which makes it “quite difficult to be expert in even one of those fields, let alone expert enough in both that you can translate and find connections between them.”
(Read more: Neuron, The Verge, “Google’s Intelligence Designer,” “Can This Man Make AI More Human?”)