Posted May 09, 2017
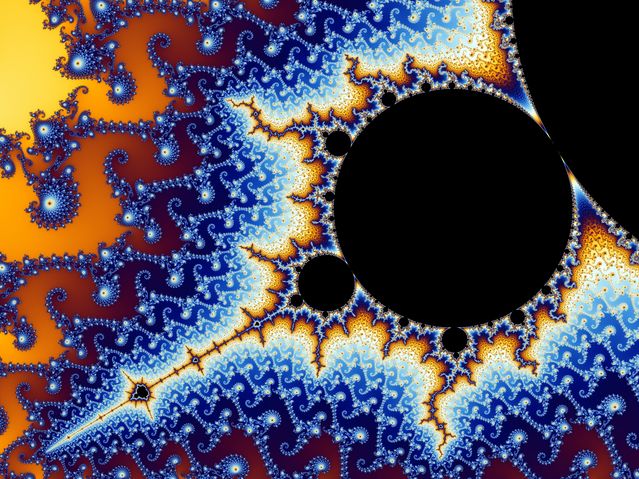
Source: Wikimedia Commons user Wolfgangbeyer
Can we learn everything about the brain by studying individual brain cells?
It started with a simple equation. In 1980, a mathematician named Benoit Mandelbrot working for IBM plotted the behavior of points on a plane using a computer. When the plane was colored by the results, a whimsical world emerged: infinitely branching scepters and spirals, never ending chasms, endless tentacles growing from heart-shaped bulbs. It appears as something from the final trippy minutes of Kubrick's 2001: A Space Odyssey, only much stranger, like a tie-dye painted by insane space aliens.
The Mandelbrot set shows complexity no matter how far we zoom in.
Source: Wikimedia Commons
Almost none of the complexity of the eponymous Mandelbrot set is readily obvious from the equation Benoit Mandelbrot plotted. Pick a pair of numbers, one real and one imaginary. Now multiply this pair by itself, many many times, and count the number of iterations it takes to exceed a certain magnitude, or distance from zero. Color each coordinate pair on the plane according to the number of iterations that point took to grow above the threshold. And viola! Complexity is born.
The shocking depth of complexity found in the Mandelbrot set may teach neuroscientists a lesson about emergent properties. Emergent properties are crucial to understanding complexity and the brain. Unlike simple phenomena, like the swinging of a pendulum, emergent properties such as intelligence and consciousness cannot be understood by merely studying simple parts of a system. Even holding the rulebook, in the case of Mandelbrot, may not readily show how the rules result in complexity. Why does squaring each number and adding back the result create such a beautifully complex pattern? Why does a particular pattern of neural connections allow for language and intelligence? To be sure, mapping cells and their synaptic connections to other cells in the brain has value. If nothing else, such maps outline which communication routes are possible. But this alone is not enough.
Closely related to emergent properties is the concept of self-organization. This is the idea that new phenomena can result from interactions between parts, with no one part leading or controlling the system. Consider the tiny worm C. elegans. Mapping all 302 neurons and synapses in the adult hermaphrodite worm should, by the opposing logic of reductionism, turn the scientist into a prescient wizard who can foresee how the worm responds to every possible stimulus. And yet, such knowledge has lead to only modest insights into C. elegans' behavior. Does this suggest that we still don't fully know the rules for how these neurons interact? Or is the simulation still not detailed enough?
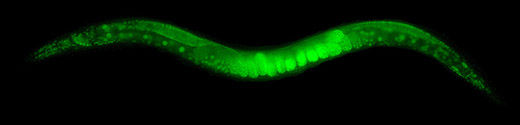
The roundworm C. elegans. Adult hermaphrodites have exactly 302 neurons.
Source: Wikimedia Commons/Dan Dickinson, Goldstein lab, UNC Chapel Hill
Sometimes we need more firepower. If we have enough powerful computers, this reasoning goes, a simulation will show us how every wiggle and breath results from each poke and prod. Such is the justification for the Human Brain Project (HBP), an undertaking co-funded by the European Union that has inherited goals from Switzerland's Blue Brain project. Lead by neuroscientist Henry Markham at the Swiss Federal Institute of Technology in Lausanne, HBP aspires to run a massive simulation of a human brain using the vast firepower of supercomputers across Europe. Not the least of these is an IBM blue gene supercomputer performing nearly six quadrillion floating point operations per second!
In the case of the Mandelbrot set, computers were the key to unlocking complexity—without their laborious firepower, it is likely that no human would ever see the haunting patterns that emerge from a simple equation. But for an emergent property to be simulated by a computer, the complete rulebook must be known. As we discover new molecules and developmental trends in the brain, our humility grows with our knowledge. Are we actually ready to build a computer model of the human brain when, as recently as several years ago, a widely accepted model of neural connections in the adult brain known as the tripartite synapse was found to be wrong? And there is still some disagreement among neuroscientists on questions as basic as how and where memories are stored in the brain. Other gaps in our knowledge—such "orphan" receptors whose neurotransmitter parents have not yet been discovered—underscore the possible hubris of such a moonshot level undertaking.
It’s important to emphasize that even small discoveries of this sort matter. Small causes may have big effects. This concept, known as nonlinearity, underlies complex systems. In Mandelbrot's case, changing the position of a point on the plane by a hair may completely alter its color or magnitude. In the brain's case, slightly adjusting the resting voltage of neurons may completely alter their collective activity. Nonlinear interaction between parts is central to self-organization.
In the Mandelbrot set, patterns on all scales exists, even if the observer zooms in for infinity. While the brain does not exhibit a truly infinite range of complexity, it does exhibit structure and activity over a vast range of different scales of space and time. Complex connectivity patterns are observed from microscopic synapses to the whole-brain scale. This facet of brain complexity urges us not to build our understanding of the brain only on cells, but all relevant scales. Indeed, the "functional unit" of the nervous system is sometimes identified as the neuron, but also as larger structures known as cell assemblies and neocortical columns.
Markham has closed a TED talk by suggesting his model brain might one day speak to humans through a hologram. Herculean goals of simulating consciousness or otherwise biting off more than the project can chew have been criticisms of HBP. But if we cannot understand emergent properties through vast computer simulations like HBP, how can we understand the brain? Is reverse engineering the brain possible?
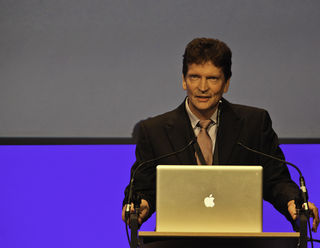
Henry Markram
Source: Flickr user cea+
A true reverse engineering approach requires understanding the brain on its most abstract level. Such holistic understanding transcends knowing that a gene or brain region is needed for memory or cognition—it explains how and why. A paper published in the journal Neuron in February calls for neuroscientists to consider how a circuit in the brain could or should work before dissecting it with a plethora of tools, just as one needs to understand such concepts as aerodynamics and lift before studying a bird’s wing. This idea, which originated with the late neuroscientist David Marr, implies that HBP first needs a theory for how language or consciousness could emerge from neurons and synapses before blindly simulating billions of them.
Until we know how and why a certain pattern of activity or piece of brain tissue is needed for behavior, we can't really claim that we understand the brain. In the meantime, there will always be room for theoreticians outside the lab to ponder our behaviors and ask what biological machinery could beget such complexity. The foundation of neuroscience need not be merely single cells, but also great ideas.
References
Bullmore, E., & Sporns, O. (2009). Complex brain networks: graph theoretical analysis of structural and functional systems. Nature Reviews Neuroscience, 10(3), 186-198.
Jabr, F. (2012). The connectome debate: is mapping the mind of a worm worth it. Scientific American,(October 2, 2012).
Jacbonson, R. (2015). Memories May Not Live in Neurons’ Synapses. Scientific American, (April 1, 2015).
Krakauer, J. W., Ghazanfar, A. A., Gomez-Marin, A., MacIver, M. A., & Poeppel, D. (2017). Neuroscience needs behavior: correcting a reductionist Bias. Neuron, 93(3), 480-490.
Theil, S. (2015). Why the Human Brain Project went wrong—and how to fix it. Scientific American, 313(4).