Researchers give AI the ability to simulate the anticipated needs and actions of others
By Chris Baraniuk on August 17, 2018
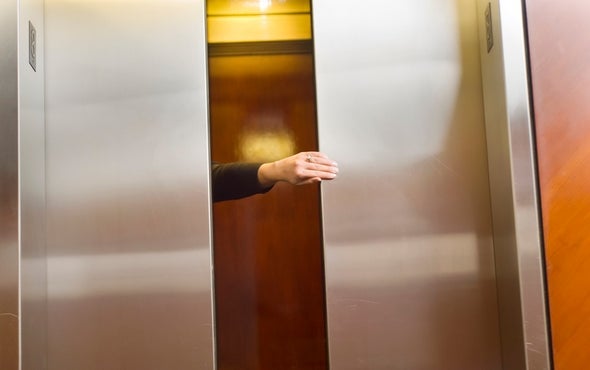
Imagine standing in an elevator
as the doors begin to close and suddenly seeing a couple at the end of
the corridor running toward you. Even before they call out, you know
from their pace and body language they are rushing to get the same
elevator. Being a charitable person, you put your hand out to hold the
doors. In that split second you interpreted other people’s intent and
took action to assist; these are instinctive behaviors that designers of
artificially intelligent machines can only envy. But that could
eventually change as researchers experiment with ways to create
artificial intelligence (AI) with predictive social skills that will
help it better interact with people.
A bellhop robot of the future, for example, would ideally be able to anticipate hotel guests’ needs and intentions based on subtle or even unintentional cues, not just respond to a stock list of verbal commands. In effect it would “understand”—to the extent that an unconscious machine can—what is going on around it, says Alan Winfield, professor of robot ethics at the University of West England in Bristol.
Winfield wants to develop that understanding through “simulation theory of mind,” an approach to AI that lets robots internally simulate the anticipated needs and actions of people, things and other robots—and use the results (in conjunction with pre programmed instructions) to determine an appropriate response. In other words, such robots would run an on-board program that models their own behavior in combination with that of other objects and people.
“I build robots that have simulations of themselves and other robots inside themselves,” Winfield says. “The idea of putting a simulation inside a robot… is a really neat way of allowing it to actually predict the future.”
“Theory of mind” is the term philosophers and psychologists use for the ability to predict the actions of self and others by imagining ourselves in the position of something or someone else. Winfield thinks enabling robots to do this will help them infer the goals and desires of agents around them—like realizing that the running couple really wanted to get that elevator.
This differentiates Winfield’s approach from machine learning, in which an AI system may use, for example, an artificial neural network that can train itself to carry out desired actions in a manner that satisfies the expectations of its users. An increasingly common form of this is deep learning, which involves building a large neural network that can, to some degree, automatically learn how to interpret information and choose appropriate responses.
A simulation-based approach relies on a pre programmed internal model instead. Winfield describes the simulation theory of mind system as using a “consequence engine.” In other words, a robot equipped with the system can answer simple “what if” questions about potential actions. If it simulates turning left, it might, for instance, detect that it would bump into a nearby wall. To make this prediction possible, the robots are pre programmed with a basic grasp of physics so that they understand what happens when objects collide. Winfield describes his robots as having a little bit of “common sense.”
For the moment, robots can only use simulation theory of mind in relatively simple situations. In a paper published in January , Winfield and his colleagues described an experiment in which a robot was designed to move along a corridor more safely ( that is, without bumping into anything) after being given the ability to predict the likely movements of other nearby robots. This is not a new capability for a robot, but in this case Winfield’s machine simulated the consequences of its own collision- avoidance strategies to make sure they would be safe. Winfield acknowledges in his study that this work is still in its nascent stages and “far from a complete solution.” For example, it took his behavior-guessingrobot 50 percent longer to traverse the corridor than when it proceeded directly to the other side without trying to anticipate another robot’s actions. Still, he proposes “simulation-based internal modeling as a powerful and interesting starting point in the development of artificial theory of mind.”
Winfield also concedes that theory of mind is still not well understood in people. Scientists have incomplete knowledge of the human brain’s “neurological or cognitive processes that give rise to theory of mind,” according to a study Winfield published in Frontiers in Robotics and AI in June. Yet he believes a full understanding of these processes is not necessary to develop AI that can perform a very similar function.
One major potential advantage of simulation theory of mind is that it may help robots become more communicative with humans—a feature that will become increasingly important as automation makes ever more inroads into human life, Winfield says. Some people might, for example, want a robot to explain its actions after the fact—something AI is generally unable to do, because the inner workings of a deep- learning artificial neural network are highly complex and may leave humans largely out of the decision-making process. And what about robots that assist elderly or ill people? Ideally such a machine could spontaneously give a warning to an elderly person, announcing that it is about to approach, to avoid alarm or confusion. Think of a nurse saying, “I’m just going to give you another pillow so you can sit up and take your medicine.” It is the difference between a robot that simply acts and one that justifies its actions before taking them.
Researchers are trying to develop machine-learning systems that explain their decision-making in human language. One basic model, for example, has an AI program determine whether an image depicts a healthy meal and explain its answer: “no” because the image includes a hot dog, or “yes” because it detects the presence of vegetables. But such programming is in its early stages and far from commonplace.
Henny Admoni, an assistant professor at Carnegie Mellon University’s Robotics Institute who was not involved in the new studies, agrees this kind of capacity would be useful. “The benefit of something like simulation theory, the way Winfield implements it, is that there is an explanation that the system can generate about what it has learned or why it did something,” Admoni says.
People will find it easier to trust a machine that can more accurately and clearly explain itself, according to Julie Carpenter, a research fellow with the Ethics and Emerging Sciences Group at California Polytechnic State University. “You have to believe that the other entity has similar goals that you do—that you’re working towards the same goal,” says Carpenter, who was not involved in Winfield’s research.
Now that Winfield has built machines that carry out simple actions determined by internal simulations, his next step is to give these robots the ability to verbally describe their intended or past actions. A good test will be if one robot can listen to statements of intent made by another robot and correctly interpret these statements by simulating them. That process would involve one robot verbally describing an action—“I’m going to hold the elevator door,” for example—and the other robot hearing this information before internally simulating the action and consequence: the doors are kept open.
If they can understand one another in such a way, they are in theory one step closer to understanding us, Winfield says— “I’m very excited by that experiment.”
A bellhop robot of the future, for example, would ideally be able to anticipate hotel guests’ needs and intentions based on subtle or even unintentional cues, not just respond to a stock list of verbal commands. In effect it would “understand”—to the extent that an unconscious machine can—what is going on around it, says Alan Winfield, professor of robot ethics at the University of West England in Bristol.
Winfield wants to develop that understanding through “simulation theory of mind,” an approach to AI that lets robots internally simulate the anticipated needs and actions of people, things and other robots—and use the results (in conjunction with pre programmed instructions) to determine an appropriate response. In other words, such robots would run an on-board program that models their own behavior in combination with that of other objects and people.
“I build robots that have simulations of themselves and other robots inside themselves,” Winfield says. “The idea of putting a simulation inside a robot… is a really neat way of allowing it to actually predict the future.”
“Theory of mind” is the term philosophers and psychologists use for the ability to predict the actions of self and others by imagining ourselves in the position of something or someone else. Winfield thinks enabling robots to do this will help them infer the goals and desires of agents around them—like realizing that the running couple really wanted to get that elevator.
This differentiates Winfield’s approach from machine learning, in which an AI system may use, for example, an artificial neural network that can train itself to carry out desired actions in a manner that satisfies the expectations of its users. An increasingly common form of this is deep learning, which involves building a large neural network that can, to some degree, automatically learn how to interpret information and choose appropriate responses.
A simulation-based approach relies on a pre programmed internal model instead. Winfield describes the simulation theory of mind system as using a “consequence engine.” In other words, a robot equipped with the system can answer simple “what if” questions about potential actions. If it simulates turning left, it might, for instance, detect that it would bump into a nearby wall. To make this prediction possible, the robots are pre programmed with a basic grasp of physics so that they understand what happens when objects collide. Winfield describes his robots as having a little bit of “common sense.”
For the moment, robots can only use simulation theory of mind in relatively simple situations. In a paper published in January , Winfield and his colleagues described an experiment in which a robot was designed to move along a corridor more safely ( that is, without bumping into anything) after being given the ability to predict the likely movements of other nearby robots. This is not a new capability for a robot, but in this case Winfield’s machine simulated the consequences of its own collision- avoidance strategies to make sure they would be safe. Winfield acknowledges in his study that this work is still in its nascent stages and “far from a complete solution.” For example, it took his behavior-guessingrobot 50 percent longer to traverse the corridor than when it proceeded directly to the other side without trying to anticipate another robot’s actions. Still, he proposes “simulation-based internal modeling as a powerful and interesting starting point in the development of artificial theory of mind.”
Winfield also concedes that theory of mind is still not well understood in people. Scientists have incomplete knowledge of the human brain’s “neurological or cognitive processes that give rise to theory of mind,” according to a study Winfield published in Frontiers in Robotics and AI in June. Yet he believes a full understanding of these processes is not necessary to develop AI that can perform a very similar function.
One major potential advantage of simulation theory of mind is that it may help robots become more communicative with humans—a feature that will become increasingly important as automation makes ever more inroads into human life, Winfield says. Some people might, for example, want a robot to explain its actions after the fact—something AI is generally unable to do, because the inner workings of a deep- learning artificial neural network are highly complex and may leave humans largely out of the decision-making process. And what about robots that assist elderly or ill people? Ideally such a machine could spontaneously give a warning to an elderly person, announcing that it is about to approach, to avoid alarm or confusion. Think of a nurse saying, “I’m just going to give you another pillow so you can sit up and take your medicine.” It is the difference between a robot that simply acts and one that justifies its actions before taking them.
Researchers are trying to develop machine-learning systems that explain their decision-making in human language. One basic model, for example, has an AI program determine whether an image depicts a healthy meal and explain its answer: “no” because the image includes a hot dog, or “yes” because it detects the presence of vegetables. But such programming is in its early stages and far from commonplace.
Henny Admoni, an assistant professor at Carnegie Mellon University’s Robotics Institute who was not involved in the new studies, agrees this kind of capacity would be useful. “The benefit of something like simulation theory, the way Winfield implements it, is that there is an explanation that the system can generate about what it has learned or why it did something,” Admoni says.
People will find it easier to trust a machine that can more accurately and clearly explain itself, according to Julie Carpenter, a research fellow with the Ethics and Emerging Sciences Group at California Polytechnic State University. “You have to believe that the other entity has similar goals that you do—that you’re working towards the same goal,” says Carpenter, who was not involved in Winfield’s research.
Now that Winfield has built machines that carry out simple actions determined by internal simulations, his next step is to give these robots the ability to verbally describe their intended or past actions. A good test will be if one robot can listen to statements of intent made by another robot and correctly interpret these statements by simulating them. That process would involve one robot verbally describing an action—“I’m going to hold the elevator door,” for example—and the other robot hearing this information before internally simulating the action and consequence: the doors are kept open.
If they can understand one another in such a way, they are in theory one step closer to understanding us, Winfield says— “I’m very excited by that experiment.”
ABOUT THE AUTHOR(S)