Angel investor + VC in high growth startups @gsvcap
I
wouldn’t do Professor Pedro Domingos justice by trying to describe his
entire book in this blog post, but I did want to share one core thought
as I reflect on his book.
In the world's top research labs and universities, the race is on to invent the ultimate learning algorithm: one…www.amazon.com
Domingos’ core argument is that machine learning needs an unifying theorem, not unlike the Standard Model in physics or the Central Dogma
in biology. He takes readers through a historical tour of artificial
intelligence and machine learning and breaks down the five main schools
of machine learning (below). But he argues that each has its limitations
and the main goal for current researchers should be to discover/create
“The Master Algorithm” that has the ability to learn any concept aka act
as a general purpose learner.

As with any great book, it leads to more questions than answers. My main question, as applied to startups, is this:
What’s the speed at which machine learning is improving?
Why is this an important question?
For the past several decades, the category defining companies from Intel to to Apple to Google to Facebook have benefited from 2 core unifying theories of technology.
First, Moore’s Law
created the underlying framework for the speed at which computing power
increases (doubling every two years or so) that has directly enabled a
generation of products. Products that were at first bulky and expensive,
such as room-sized mainframes, were able to ride Moore’s Law and become
smaller and cheap, leading to mass products like phones and smart
watches.
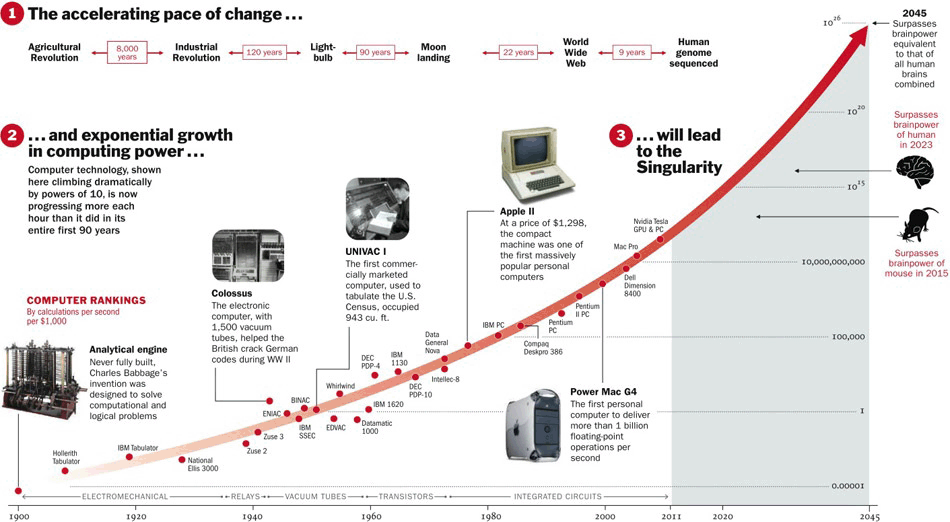
Second, Metcalfe’s Law governed the value of a network of users (n(n
− 1)/2) that has enabled a generation of Internet services to
effectively serve the majority of the world’s Internet population. As
more users join a network, their value grow exponentially while costs
generally grow linearly. This incentivizes even more users and the
flywheel is set in motion.
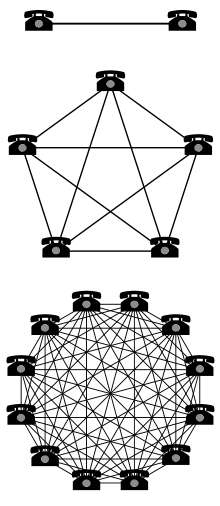
So now the question is…is there a third “Law” that governs the speed of improvement of machine learning.
In Lee Kai-Fu’s (李开复) commencement speech at Columbia, he gives hints at this.
In speaking about his investments in now publicly listed Meitu
and two other AI investments, he notes that in all three cases, the AI
technology underlying the startups went from essentially not useful to
indispensable.
The three software companies I mentioned earlier, when they were first launched: often made people uglier, lost millions in bad loans, and thought I was some talk show celebrity. But given time and much more data, their self-learning made them dramatically better than people. Not only are they better, they don’t get tired nor emotional. They don’t go on strike, and they are infinitely scalable. With hardware, software, and networking costs coming down, all they cost is electricity.
In god we trust, all others bring data…
To put some data behind it, if we look at the ImageNet Challenge,
AI image recognition technology has improved 10X from 2010 to 2016,
catalyzed by the introduction of deep learning methods in 2012.
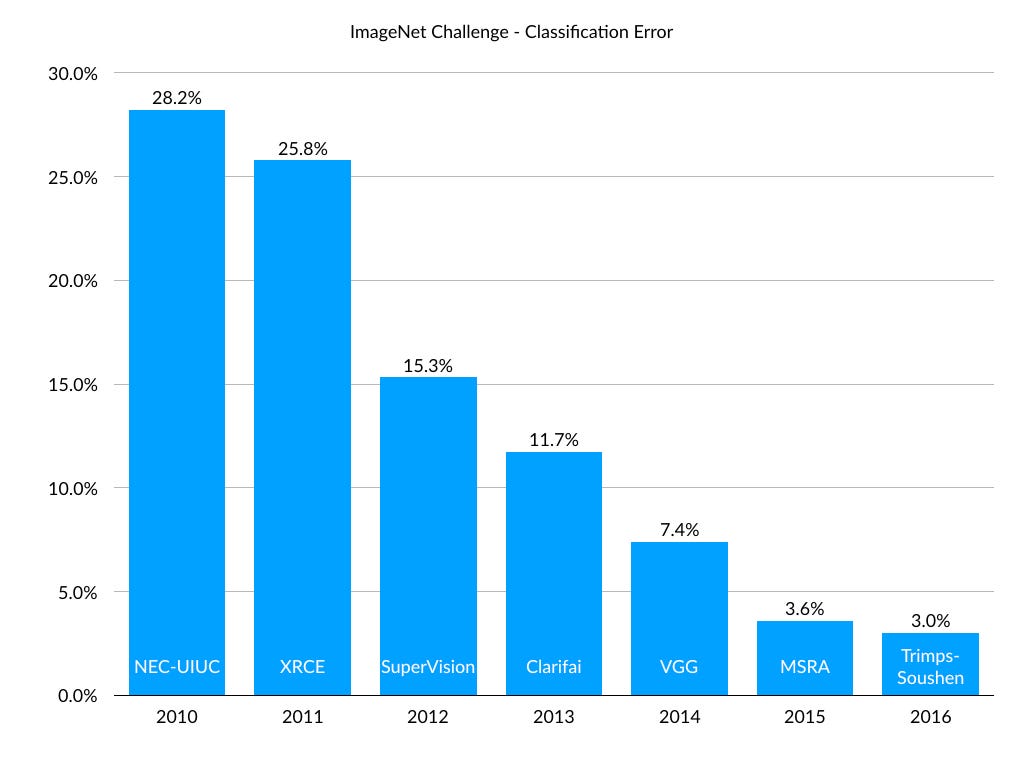
On
the backs of this “Law” of machine learning improvement, we’ll see a
Cambrian explosion of new products and services that fits Kai-Fu’s
description of products that are at first flawed, but with time and
data, become essential and, for all practical purposes, perfect.
Questions, not answers
The
ImageNet Challenge and image recognition is just one application of AI
so it doesn’t give us enough to say what the “Law” of machine learning
improvement is. I can’t say AI is doubling in intelligence every 18 to
24 months or that AI gets exponentially better by a factor of n(n − 1)/2 with each data point.
But I do think a particular “Law” governing the rate at which AI is improving exists and I can’t wait for someone in the field to articulate (or solve) it.
Because
understanding the speed at which artificial intelligence is getting
more intelligent will allow us to understand the third major
foundational wave, in addition to Moore’s and Metcalfe’s Law, that will
bring us the dominant companies and the brilliant products of the Age of
AI.
Join 25,000+ people who read the weekly 🤖Machine Learnings🤖newsletter to understand how AI will impact the way they work.